chapter 1: weather risk management for agriculture by joanna syroka1 1. introduction to weather risk ===============================
Chapter 1: Weather Risk Management for Agriculture
By Joanna Syroka1
1. Introduction to Weather Risk
===============================
The emerging weather risk market offers new risk management tools and
opportunities for agriculture. The aim of this chapter is to
illustrate how an end user in the agricultural industry could use a
market-based solution to mitigate the financial impact of weather on
its business operations. The chapter draws information from the wealth
of literature written on the subject of weather risk management, with
an aim to provide the reader with a step-by-step guide to how weather
risk management instruments could be used and developed for the
agricultural sector. The chapter is divided into four sections.
Section 2 will focus on the key steps required to structure a weather
risk management solution, from identifying the risk to execution.
Section 3 will focus on the pricing of weather risk management
instruments, giving a brief overview of how the weather market
approaches and values weather risk and the implication this has for
the end user. Section 4 will focus on the pre-requisites for weather
risk management instruments, namely the weather data used to construct
weather indices and to settle contracts. This section will also touch
upon data cleaning and analysis that must be considered when pricing
and structuring a potential transaction. Section 5 will summarize the
chapter and reference sources for further reading on weather risk
management.
The Financial Impact of Weather
-------------------------------
Weather risk impacts individuals, corporations and governments with
varying degrees of frequency, severity and cost. Around the world
people face the vagaries of the weather on a daily basis. The media
continually reports catastrophic weather events – floods, hurricanes
and droughts – that impact individuals’ property, health and lives.
Consequently, governments are also financially exposed to weather
risk. They are called upon to provide direct financial, nutritional
and housing support to their citizens in the event of weather-related
disasters and must increase spending for rehabilitation and
reconstruction of infrastructure and assets as a result of damage
incurred. Moreover the economy of a country is also at risk to weather
through business interruption, supply shocks, diversion of domestic
investment from productive activities to mitigation of disasters’
impacts and, for some countries, a reduction in foreign investment in
the aftermath of an extreme weather-related event. For example, with a
death toll exceeding 30,000 (14,000 in France alone), the heat wave
and drought across Europe in the summer of 2003 was the worst natural
disaster in the region in the past 50 years. Aside from the human
impact, the extreme conditions particularly affected the agriculture,
forestry and energy sectors: the total financial impact was estimated
to exceed €13 billion - the financial impact on agriculture and
forestry in France was estimated to be €4 billion alone. As a result
the extreme summer heat appears to have contributed to a weak European
GDP2 in the third quarter of 2003.
While often such effects are reversible and short-term, the impact on
the economy of a poor country can be significant and long lasting.
Between 1997 and 2001, the average damage per natural disaster in
low-income countries was five percent of GDP3. Evidence from sixteen
Caribbean countries shows, for example, that one percentage point of
GDP in direct damage from natural disasters can reduce GDP growth by
half a percentage point in the same year4. Furthermore the
humanitarian cost of weather-related disasters is also greater in the
developing world: approximately 80%5 of all fatalities due to weather
disasters from 1980-2003 occurred in the “uninsured world”, comprised
predominantly of low-income countries.
However, even non-catastrophic weather events have a financial impact.
The U.S. Department of Commerce estimates that nearly one-third of the
U.S. economy, or $1 trillion6, is modulated by the weather and that up
to 70% of all U.S. companies are weather sensitive. Weather risk can
impact a business through its overall profitability or simply through
the success or failure of an initiative as a consequence of the
weather. Like governments, businesses can face both demand and supply
driven weather risk. Energy companies, for example, can be exposed to
demand driven weather risk. For instance, in the event of a warmer
than average winter, gas companies, in particular those who deal with
domestic customers, face a potential drop in gas sales as customers do
not use as much gas as expected to heat their homes. Therefore even if
the company has adhered to prudent price risk management practices by
protecting their sales margin from fluctuations in the gas supply
price, a drop in sales volume from expected levels can still have a
significant impact on budgeted revenues simply through weather-driven
demand fluctuations. A supply-side example of weather risk can be
found in the construction industry. Cold and wet weather conditions
can impact construction progress as building materials have specific
weather requirements, for example concrete cannot be poured in wet or
below-freezing conditions. The contractor therefore must assume this
supply-driven weather risk, which can significantly delay a
construction project and result in hefty penalties if the project is
not completed on-schedule. This recent excerpt from the Central New
Jersey Home News Tribune illustrates the example:
“The extension of Route 18 into Piscataway, which had been discussed
for more than four decades and has frustrated motorists since
construction began in June 2002, may not be completed until fall 2005
because of adverse weather conditions. The first phase of the project
-- to provide a River Road overpass and an extension of Metlars Lane
from the John A. Lynch Sr. Bridge to Hoes Lane -- had been scheduled
to be completed by November. But the project's construction company,
Slattery Skanska Inc. of Whitestone, N.Y., hampered by a wet spring
and summer and sustained cold weather this winter, has applied for a
delay, according to Department of Transportation spokesman Mike Horan.
"A lot of our projects have been hampered by the weather," said Horan.
Horan explained that when the ground is frozen a proper bed cannot be
laid for roadways, and asphalt cannot be used until the temperature
remains above freezing. … Horan explained that the application for a
delay beyond November will be studied by the DOT. Unless a delay is
granted, the construction company could face penalties, according to
Horan.”7
Weather has traditionally been the scapegoat in business for poor
financial performance8. Annual reports, financial statements and press
releases frequently contain declarations such as “[c]ooling degree
days were 21 percent below last year’s quarter and 16 percent below
normal. The effects of milder weather compared with last year had a
negative impact on EBIT of about $35 million for the quarter.”9, “4
cents per share [decline] for lower gas deliveries due to warmer
weather in the fourth quarter of 2003”10, “[d]ifferences in heating
demand caused by weather variations between years resulted in a $13
million margin decrease as warmer-than-normal temperatures were
experienced during both years. During 2003, operating margin was
negatively impacted $32 million by the weather, while in 2002 the
negative impact was $19 million.”11 and “Europe's performance
continued to be impacted by unfavorable summer weather with volume
down 12 percent in the third quarter and year-to-date volume down 6.5
percent.”12 Given such examples it is not surprising that the
financial community has begun to seek practical solutions to
controlling the financial impact of weather. For example, Centrica
Plc, one of the largest domestic gas supplier in the UK, is one of a
number of utilities that has chosen to manage its weather risk in
order to “protect the company against variability in earnings of its
gas retail business due to abnormal winter temperatures in the UK”13
and has been doing so since 1998. London-based Corney and Barrow Wine
Bars Limited has deployed several weather hedges to provide financial
protection against cool summers resulting in poor customer patronage,
“After the exceptional summer of 2003 Corney and Barrow was keen to
secure protection against the possibility of the reverse experience
[in 2004]”14. Blaming lost revenues or increased expenses on weather
may no longer be an excuse accepted by stakeholders. With the
emergence of a market for weather risk management products, a business
can now be protected from such ancillary risks that create
unpredictable earnings streams. Just as interest rate and currency
risks are currently managed through market-based solutions, CFOs can
now neutralize weather risks that increase business uncertainty,
allowing a company to focus on its core business and to protect
earnings per share forecasts and growth.
For instance, in September 2003 Northern Foods, a UK-based food
company, announced that its second-half profits would be
“significantly lower” than last year. The group blamed unseasonably
hot weather that had reduced demand for meat pies and damaged harvests15.
The profit warning prompted market analysts to cut their forecasts for
the food group’s full-year results, triggering a 15 per cent fall in
Northern Foods shares and coinciding with the resignation of the
company’s CEO. As financial analysts are beginning to highlight the
impact of weather on operations and corporate earnings they are also
beginning to recognize the advantage of weather risk management, as
echoed by a UBS analyst, “Earnings surprises are not liked by the
market...I believe weather futures will be one of the fastest growing
financial instruments over the next decade.”16 It is clear a company
that actively manages its weather risk is in a stronger position than
one that does not.
The Weather Market
In 1997 a formal weather risk market was born through the first open
market derivative transaction indexed to weather in the United States.
Motivated by the deregulation of the energy industry which led to the
break-up of regulated monopolies in electricity and gas supply, the
nascent weather market responded to the need for energy companies to
increase operational efficiency, competitiveness and shareholder
value. In 1996, the Kansas-based energy company Aquila entered into a
transaction with New York-based Consolidated Edison that combined
temperature and energy indicators, protecting the latter against a
cool August that would reduce power sales. However the first
publicized transaction in 1997 was between energy companies Koch
Energy and Enron. Additional deals soon followed with other energy
market participants wanting protection against risks, primarily
temperature, associated with volumetric fluctuations in energy.
In the context of the weather market, weather risk is defined as the
financial exposure that an entity – an individual, government or
corporation – has to an observable weather event or to variability in
a measurable weather index that causes losses to either property or
profits. All weather contracts are based on the actual observations of
weather at one or more specific weather stations. In contrast to
traditional insurance products, where recovery is determined on a
loss-adjustment basis, weather risk management products – packaged as
either (re)insurance or derivatives – are primarily settled off of the
same index that has been determined to cause losses. Weather-indexed
risk management instruments therefore provide financial protection
based on the performance of a specified weather index in relation to a
specified trigger. The design of a verifiable and objective index
which correlates closely with the underlying weather impact not only
streamlines traditional insurance practices but also creates
opportunities to manage non-catastrophic – or near-the-mean – risk
that impacts a company’s earnings. Previously, traditional insurance
products primarily dealt with physical losses of assets (e.g. property
and infrastructure) that were associated with low frequency/high
severity catastrophic weather events.
In 2001, the Weather Risk Management Association (WRMA) – the industry
body – commissioned PricewaterhouseCoopers (PWC) to conduct a survey
of weather risk contracts executed among WRMA members and survey
respondents from October 1997 to March 2001, and since then on an
annual basis. Since 1997, the survey has shown that over US$20 billion
has been transacted through the weather risk market to date – the
market has grown to around US$4.6 billion outstanding risk for the
year April 2003 – March 200417 (Figure 1), although some believe this
to be an underestimate18. There is active trading in U.S., European
and Japanese cities (Figure 2) with a few transactions outside these
three main trading hubs, most notably agricultural transactions in
Mexico, India and South Africa. The market has also evolved to include
non-energy applications. Survey respondents, when asked to list
requests received from potential end users of weather risk management
products, identified end users in the retail, agriculture, transport
and leisure and entertainment industries (Figure 3), although energy
still contributes approximately 56% of the potential weather risk
management end user market. As a result of this expansion the market
has also broadened its product offering to include transactions on
non-temperature indices19 such as rainfall, wind and snow (Figure 4).
One of the most notable transactions in the market was that of SFB
Groep20, a Dutch construction workers’ union and employers’
federation, through Dutch investment bank ABN AMRO in 2001. The body,
who have re-entered the market since, bought multi-year protection to
provide compensation in the case of cold weather halting daily
construction work, with a notional value of several hundreds of
millions of Euros. With the coming deregulation of energy markets in
continental Europe and Japan and with increased focus on shareholder
value and risk management in the financial markets, the weather market
is forecast to grow further.
Today the key market participants include (re-)insurers, investment
banks and energy companies. (Re)insurers and investment banks provide
weather risk management products to end user customers – such as
Corney and Barrow Wine Bars Limited, Centrica Plc and the Dutch
construction workers’ union and employers’ federation – and form the
primary market; all three participate in a secondary market in which
players transfer weather risk through over-the-counter (OTC) financial
transactions and exchange-based derivative contracts on the Chicago
Mercantile Exchange21 (CME) amongst themselves to diversify and hedge
their portfolios. In addition to core weather market participants,
professional investors, such as alternative risk hedge funds, are also
becoming interested in weather risk and are beginning to source excess
risk from the primary weather market as well as participating in the
secondary market through the CME. Weather is an uncorrelated risk that
can enhance their portfolio positions and differentiate them from
other funds.
Weather risk management is also being introduced to the developing
world through the work of organizations such as the World Bank’s
Commodity Risk Management Group (CRMG) and the United Nation’s World
Food Programme (WFP). The World Bank was involved in the first
index-based weather risk management program in India in June 2003, and
is currently working on several projects around the world. The small
pilot program was launched by Hyderabad-based micro-finance
institution BASIX and Indian insurance company ICICI Lombard in
conjunction with CRMG, where 230 groundnut farmers in Andhra Pradesh
bought weather insurance to protect against low monsoon rainfall22.
Currently the WFP, in conjunction with the World Bank, are
investigating the feasibility of weather-based insurance as a
reliable, timely and cost-effective way of funding emergency
operations in countries such as Ethiopia23. Work is also underway to
see if developing country governments in southern Africa can benefit
from weather risk management products and strategies.24 The global
weather-risk market is particularly interested in these types of
transactions, as they provide much sought after diversification to
their books through new locations and risks.
Weather Risk and Agriculture
One of the most obvious applications of weather risk management
products, be it weather insurance or weather derivatives, is in
agriculture and farming. Indeed 13%25 of the end user requests in the
weather market are now focused on the agricultural sector (Figure 3).
Weather impacts many aspects of the agricultural supply and demand
chain. From the supply side, weather risk management can help control
both production or yield risk and quality risk.
Technology plays a key role in production risk in farming. The
introduction of new crop varieties and production techniques offers
the potential for improved efficiency, however agriculture is also
affected by many uncontrollable events that are often related to
weather – including excessive or insufficient rainfall, hail, extreme
temperatures, insects and diseases – that can severely impact yields
and production levels. Countless examples can be given on the impact
of cold temperatures on deciduous fruit26, deficit rainfall on wheat27,
excess rainfall on potato yields28 and even temperature stress on
cattle and thus dairy production29. In 2001 California’s wine revenue
fell by over $200 million, which was largely attributed to frost
damage of wine grapes in April of that year30. In 2003, 64% of
Ukraine’s winter wheat crop was destroyed due to winterkill
temperatures and 40-50% of northeastern England’s oil rapeseed crop
was lost to due excessive rain at harvest in August 2004. The costs
associated with drops in expected or budgeted production due to such
uncontrollable factors can have a significant impact on a producer’s
revenues and contractual obligations, as reflected in an financial
statement of JG Boswell, the largest U.S. cotton grower, “Both 1997
and 1998 fiscal results were impacted by extremely harsh winter
patterns that flooded over 41,000 acres of the Company’s Corcoran
farming districts causing a decrease of $1,000 per acre or $41 million
in gross revenues. Additionally, cold and wet spring weather delayed
cotton planting by up to six-weeks which resulted in some of the worst
farming conditions management has ever seen.” A producer may seek
protection against adverse weather conditions that impact the yield of
the crop farmed.
Weather can also impact the quality, if not the absolute production
levels, of a crop. An example can be taken from the brewing industry.31
A large brewery needs a specific quality of barley for its production
of beer and contracts land for barley production in order to have
direct access to the quality of barley it needs. The key risk to the
quality of the barley produced occurs once the plant is mature where
excessive rain and humidity will cause the seed to lose weight and
discolor. In years where the crop quality is insufficient, the barley
can be used for animal feed or alcohol at a lower market value, but
the brewery will still need to purchase the barley at market prices
incurring an additional cost to the brewery – a cost that can be
insured against by the purchase of an appropriate weather risk
management product. On the demand side, weather also impacts related
agricultural products through the use of pesticides, fertilizers and
herbicides. Agricultural chemical producers, for example, can use
weather risk management instruments to hedge against the costs
associated with fluctuations in the demand for chemicals by farm
operators. Increases in pesticide sales are often related to weather
conditions, particularly accumulated Growing Degree Days32, that
impact the gestation period and hence the birth rate of pests. Cotton
boll weevil, which costs cotton producers in the U.S. $300 million a
year33, is an example of a weather sensitive pest whose numbers differ
from year to year largely due to the severity of the winter. In
extremely cold winters weevil numbers drop significantly, directly
affecting the net earnings of an agrochemical company. Chemical
producers could hedge their earnings volatility through fluctuations
in pesticide sales by purchasing a weather risk management instrument
specifically indexed to the phenology of pests their products target.
Alternatively, as part of a marketing strategy, they could offer their
clients an embedded weather option in the chemical sales contract to
provide customers protection from the cost of extra applications as a
result of weather conditions favoring pest development34.
Index-based weather insurance is a relatively new product and the use
of weather risk management products in the agricultural sector is
still in its infancy, with very few publicized transactions in the
U.S. and Europe. However there have been a number of agricultural
transactions outside of the main weather market trading hubs, most
notably in Canada (Ontario – maize; Alberta – forage), Argentina
(Sancor – dairy), South Africa (Gensec Bank – apple cooperative freeze
cover) and India (ICICI Lombard – groundnut, cotton, coriander and
orange). Given weather is one of the biggest risks faced by farmers,
weather-indexed risk management products have been suggested as a
potential alternative to the traditional crop insurance programs for
smallholder farmers in the emerging markets35. Traditional multi-peril
crop insurance programs have several problems when they are translated
from the developed world to emerging markets (see Chapter 3 for
further discussion). Most notably the high unit administration costs,
high entry barriers for farmers and difficulties of control make
traditional crop insurance schemes neither practical nor cost
effective in small-farmer economies. These new weather risk management
insurance instruments provide a viable alternative to traditional
insurance instruments, potentially offering real advantages to
households, businesses and governments in developing countries.
2. Structuring a Weather Risk Management Solution
=================================================
Developing a successful weather risk management and transfer program
for agriculture involves four essential steps:
1.
Identifying significant exposure of an agricultural
grower/producer to weather;
2.
Quantifying the impact of adverse weather on their revenues;
3.
Structuring a contract that pays out when adverse weather occurs;
and
4.
Executing the contract in optimal form to transfer the risk to the
international weather market.
Each of the steps is outlined in the following four subsections and
they are fully explored in the case studies in the next Chapter.
Identifying the Risk
====================
Identifying weather risk for an agricultural grower or producer
involves three steps: identifying the regions at risk to weather and
the weather stations that reflected that risk; identifying the time
period during which risk is prevalent; and identifying the weather
index that is the best proxy for the weather exposure. This latter
step is the most critical in designing a weather risk management
strategy based on an index. Rather than measuring the actual impact on
crop yields – or related fluctuations in demand, supply or
profitability – the index acts as a proxy for the loss experienced due
to weather and is constructed from actual observations of weather at
one or more specific weather stations.
i. Location and Duration
All weather contracts are based on the actual observations of weather
variables at one or more specific weather stations. Transactions are
based on either a single station, a basket of several stations or on a
weighted combination of readings from multiple stations. More
information on the weather station and data requirements for weather
risk management instruments will be given in Section 4. If an
individual farmer is interested in purchasing weather protection for
his particular crop, the index-based weather contract must be written
on a weather station nearest the farmer’s land in order to provide the
best possible coverage for the farmer client. A larger grower, with
several production regions, may be more interested in purchasing a
weather contract based on several weather stations that reflects the
weather conditions in all areas covered by the business. The grower’s
risk management strategy can be either to purchase a weather contract
on each of the identified weather stations or to purchase a single
contract on a weighted average of several stations, with the
weightings chosen to reflect the importance of the different stations
to the overall weather exposure of the business. The approach chosen
depends on the risk preferences and risk retention appetite of the
grower, although generally the latter is a cheaper and more efficient
approach. Retaining localized risks will most probably be a more
cost-effective solution than transferring them to a third-party, but
will still provide protection in situations where adverse weather
affects several regions impacting the overall production portfolio of
a producer. The latter approach will also reduce the risk of reliance
on one weather station and hence the associated issue of basis risk36,
which will be covered in Section 3.
All contracts have a defined start and end date that limit the period
over which the underlying index is calculated. This calculation period
describes the effective dates of the risk protection period during
which relevant weather parameters are measured at the specified
weather stations. For agricultural end users, the duration of the
weather contracts will be determined by the specific requirements of
their business. Obvious examples include the growing season for the
crop produced, for example April-October for corn in southern Europe,
or a specific phase in the development of a crop that is particularly
sensitive to a specific weather variable, such a rainfall during the
three-week silking and tassleing period of corn37. However the
duration of contracts have the flexibility to address individual end
user business exposures and can be weekly, monthly, seasonal and even
multi-annual. Final settlement of the weather contracts typically
occur up to 40 days after the end of the calculation period, once the
collected weather data has been cross checked and quality controlled
by the relevant data-collecting body, usually the National
Meteorological Service38.
ii. Underlying Indices
A weather index can be constructed using any combination of measurable
weather variables and any number of weather stations that best
represent the risk of the agricultural end user. Common variables
include temperature and rainfall, although transactions on snowfall,
wind, sunshine hours, streamflow, relative humidity and
storm/hurricane location and strength are also possible and are
becoming more frequent. In contrast with energy, where the
relationship between energy demand and weather is more transparent and
linked primarily to temperature, in agriculture the relationship
between crop yields or pesticide use is generally more complex, albeit
still quantifiable.
For example, the normal process for designing an index-based weather
insurance contract for an agricultural grower involves identifying a
measurable weather index that is strongly correlated to a crop’s yield
rather than the yield itself. After gathering the weather data, an
index can be designed by: i) looking at how the weather variables have
or have not influenced yield over time; ii) discussing key weather
factors with experts such as agro-meteorologists and farmers; and/or
iii) referring to crop growth models which use weather variables as
inputs for yield estimates; phenology models can be used to establish
how weather variations relate to pest development. A good index must
account for the susceptibility of crops to weather factors during
different stages of development, the biological and physiological
characteristics of the crop and the properties of the soil. If a
sufficient degree of correlation is established between the weather
index and yield or crop quality, a farmer or an agricultural producer
can insure his production or quality risk by purchasing a contract
that pays in the case that the specified weather event occurs (or does
not occur). The index possibilities are limitless and flexible to
match the exposure of the agricultural grower or producer, as long as
the underlying data is of sufficient quality (Section 4). Examples
weather indices for specific agricultural exposures are given below.
Although the examples are based on temperature and precipitation, the
principles apply to all weather parameters recorded by ground-based
meteorological weather stations.
Temperature
-----------
Temperature-based indices account for over 80% of the risk in the
current weather market39. Although most of these transaction are based
on indices specifically designed for the energy industry – such as
cumulative Heating Degree Day (HDD)40 values, days in which energy is
used for heating, and cumulative Cooling Degree Day (CDD)41 values,
days in which energy is used for air conditioning – temperature is
also a pertinent weather variable for agriculture. Whereas irrigation
systems can often be used to regulate soil moisture for crops,
temperature and its impact on crop yield and quality is often a more
difficult parameter to control, particularly over the course of a
growing season. Temperature indices can be based on hourly, average,
maximum or minimum temperature, either calculated on a cumulative
(e.g. growing degree day) or event driven basis (e.g. frost risk).
Growing Degree Days. Growing Degree Days (GDDs) is a common index used
in the agricultural sector, similar to HDDs and CDDs in the energy
sector. GDDs are a measurement of the growth and development of plants
(both crops and weeds) and insects during a growing season. Organisms
that cannot internally regulate their own temperature are dependent on
the temperature of the environment to which they are exposed.
Development of an organism does not occur unless the temperature is
above a minimum threshold value, known as the base temperature, and a
certain amount of heat is required to for development to move from one
stage to the next. The base temperature varies for different organisms
and is determined through research and scientific considerations. A
list of reported base temperature examples is given in Table 142.
Table 1. Reported Base Temperatures for GDD Computations for Crops and
Insects
Base Temperature (deg Celsius) for GDD Computation
Crop/Insect Example
4.44
Wheat, Barley, Rye, Oats, Flaxseed, Lettuce, Asparagus
7.22
Sunflower, Potato
10.00
Sweet Corn, Corn, Sorghum, Rice, Soy Bean, Tomato
6.67
Corn Rootworm
8.89
Alfalfa Weevil
10.00
Black Cutworm, European Corn Borer
11.11
Green Cloverworm
A GDD is calculated by the following equation:
Daily GDD = max(0, (Taverage – L)); Taverage = (Tmax + Tmin)/2 (1)
where L is the baseline temperature and Taverage is the daily mean
temperature, defined as the average of the daily maximum (Tmax) and
minimum (Tmin) temperatures. If this average is greater than the
threshold temperature L, the GDD accumulated for that day is the
threshold temperature minus the daily average temperature. If the
daily average temperature is less than the base temperature, then the
GDD for that day is zero. Adding the GDD values of consecutive days
gives the accumulated GDDs over a specific period.
However, high temperatures can also cause development of certain
organisms to cease. The underlying temperature data can be modified to
take this into account: if the daily minimum temperature is less than
the baseline it is reset to the baseline level to avoid negative
growing degree days; if the maximum temperature is greater than the
upper limit it is reset to the upper limit, indicating no growth
benefit from temperatures above that level. Once the minimum and
maximum temperatures have been modified and a new average computed,
the Modified Growing Degree Day (MGDD) value for the day is computed
by comparing the modified average with the base temperature as in
Equation 1. MGDDs are typically used to monitor the development of
corn, the assumption being that development is limited once the
temperature exceeds 30 deg Celsius. Other, more accurate methods to
calculate GDDs, include considering the relationship between the
growth rate and temperature of an organism by either estimating the
diurnal variation in temperature or using hourly temperature readings.
Accumulated GDDs are a good proxy for establishing the development
stages of a crop, weed or insect and can give an indication as to the
development and maturity of a crop or to when pesticide or herbicide
applications should be scheduled. Measuring the amount of heat
accumulated over time provides a physiological time scale that is
biologically more accurate than calendar days43; specific organisms
need different accumulated GDDs to reach different stages of
development. For example, the growing cycle of corn consists of
vegetative, reproductive and maturation phases, with more detailed
stages of development within these broad periods. Different maturity
phases require different GDD accumulations and Table 244 shows an
example of growing cycle requirements of a 2700 GDD corn hybrid.
Table 2: Growing Degree Day Requirements for Different Phenology
Stages of a 2700 GDD Corn Hybrid.
Phase
Development Stage
GDD
Planted
0
Two leaves fully emerged
200
Vegetative
Four leaves fully emerged
345
Six leaves fully emerged
475
Growing point above soil
Eight leaves fully emerge
610
Tassel beginning to develop
Tenth leaves fully emerged
740
Twelve leaves fully emerged
870
Ear formation
Fourteen leaves fully emerged
1000
Reproductive
Silks developing on ear
Sixteen leaves fully emerged
1135
Tip of tassel emerging
Silks emerging/pollen shedding
1400
Plant at full height
Kernels in blister stage
1660
Kernels in dough stage
1925
Kernels denting
2190
Maturation
Kernels dented
2450
Physiological maturity
2700
By comparing accumulated GDD totals with previous years it can be seen
if a normal amount of heat energy has been made available to a crop.
If GDDs are running behind normal, this usually means that plant
development is delayed. A late maturing crop may be at risk to frost
or other adverse weather conditions if it does not reach maturity by a
specific time. For example, at 2700 GDDs dry matter is no longer being
translocated to the grain, indicating that corn has reached
physiological maturity and is safe from freezing conditions45;
however, if the 2700 GDD limit has not been reached the crop may be
susceptible to damage if frost occurs. In general, assuming adequate
moisture supplies are available, the total GDDs received by the end of
the growing season are often related to crop yield, therefore GDDs can
be a good index for crop production. The cumulative temperature index
can be used to establish a relationship between GDDs and production
and thus ultimately with a producer’s revenues (Box 1).
Chilling Degree Hours. Another example of a cumulative temperature
based index is chilling degree hours or units. Although cold
temperatures are often detrimental to crop production they can also
been an essential requirement for certain cultivars as described by
David H. Byrne and Terry Bacon, Dept. Of Horticultural Sciences, Texas
A&M University,
“Stone fruit trees such as peaches develop their vegetative and
fruiting buds in the summer and, as winter approaches, the already
developed buds go dormant in response to both shorter day lengths and
cooler temperatures. This dormancy or sleeping stage protects these
buds from oncoming cold weather. Once buds have entered dormancy, they
will be tolerant to temperatures much below freezing and will not grow
in response to mid-winter warm spells. These buds remain dormant until
they have accumulated sufficient chilling units (CU) of cold weather.
When enough chilling accumulates, the buds are ready to grow in
response to warm temperatures. As long as there have been enough CUs
the flower and leaf buds develop normally. If the buds do not receive
sufficient chilling temperatures during winter to completely release
dormancy, trees will develop one or more of the physiological symptoms
associated with insufficient chilling: 1) delayed foliation, 2)
reduced fruit set and increased buttoning and, 3) reduced fruit
quality.”46
There are various models used to define and calculate chilling degree
units which could be used an index for a weather risk management
strategy. The three most common models are: a) the number of hours
during the winter period where the temperature is below 7.2 degrees
Celsius; b) the number of hours where the temperature is between 0 and
7.2 degrees Celsius and; c) a model that associates varying chill
units according to the actual hourly temperature, known as the Utah
model47. The first two models are simple and define a chilling unit as
one hour below or between certain temperatures. The Utah method is
more complex because it introduces the concept of relative chilling
effectiveness and negative chilling accumulation. Average monthly
temperature can also be used to estimate accumulated chilling units.
Event Based Indices. However, crop damage can also be the result of
specific or critical temperature events that can be detrimental to
yield or quality. For instance freezing conditions were reported to
have caused more than $600 million in damage to the U.S. citrus crop
in a single week of December 1998, with $300 million occurring in
Tulare County, California, alone48. Critical temperatures causing crop
damage may vary depending on the length of time that temperatures
remain below freezing as well as the variety, health and development
stage of a plant. Table 3 gives approximate critical temperatures for
a selection of crops49. Preventative and proactive measures can often
be taken to protect crops from such events, but these may have limited
impact or become more difficult for crops that are farmed in large
areas, such as cereals and grains.
Table 3: Critical Temperatures that Result in Freeze Damage to Crops
Critical Temperature for Freeze Damage
Crop Example
0 to -1 deg Celsius
Strawberries and Raspberries (blossom and fruit), Tomatoes, Cucumbers,
Melons, Peppers, Squash and Pumpkin (plants), Beans, Tobacco
-1 to -2 deg Celsius
Potatoes, Corn, Apples and Plums (blossom), Pears and Cherries
(blossom and fruit), Beans
-2 to -4 deg Celsius
Apples (fruit and buds), Blueberries, Alfalfa, Pears
For example, winter wheat yields at harvest depend to a great extent
on how well the plants survive the winter hibernation period. On the
territory of Kherson in Ukraine winter wheat winter crops have been
known to die as a result of air and therefore soil temperatures
falling below a critical level for one day or longer. These winterkill
events cause damage and death of the plants’ tillering node, “[With
little or no snow plants begin to die when] the daily minimum air
temperature drops below –16 deg C; [a crop can be completely lost if
this happens for] four days in a row or in the minimum temperature
drops below –21 deg C”50. Snow cover considerably improves conditions
of winter wheat hibernation, as the difference between air and soil
temperature increases from 0.5 to 1.1°С per centimeter of snow cover.
However, snow cover on the territory of Kherson is often unstable
hence complete winter wheat crop failure due to winterkill is a
potential risk in the southern steppe zone of Ukraine – the crop
usually dies in years with no snow cover or where the stable snow
cover appears late in winter, such as in 2003. A winterkill index,
based on days where the daily minimum temperature is less than -16 deg
Celsius, could therefore be used by a farmer to obtain protection
against such crop failure risk. For instance, a farmer could enter
into a contract where the recovery is the full value of the crop, as
expected under normal weather conditions, if the recorded daily
minimum air temperature is less than -16 deg Celsius for four or more
consecutive days at anytime during the winter period, November to
March.
Although soil temperature is perhaps a more pertinent variable for the
farmer it is not a variable that is often measured by meteorological
weather stations and therefore could not be used to design a
winterkill risk management solution. Air temperature is generally
recorded at the 2m level by National Meteorological Services, however
a combination of air temperature and snow-cover at the nearest
measuring location could be used, for example, to construct a more
accurate index reflecting the winterkill risk for wheat.
Excessive heat can also damage crop production and quality and can be
a lot more difficult to control than freezing temperatures. For
example significant losses in winter wheat harvest are very likely
when daily maximum air temperatures exceed +30°C (at the height of 2
m) at critical shaping and ripening stages of winter wheat kernels in
late spring and early summer. One such heat stress event can lead to
up to a 4% decrease in yields at harvest time51 due to excessive
drying and underdevelopment of wheat ear and kernels.
Precipitation
-------------
Precipitation, either rainfall or snowfall, can also be vital to crop
growth and development. In the example above it was clear that a
snowfall has a critical role in protecting hibernating crops from the
damaging effects of low air temperatures. However rainfall variability
is often the most critical factor in agriculture.
Deficit Rainfall and Drought. Meteorological drought is usually
defined in terms of deviations of precipitation from normal levels and
the duration of dry periods in a region. Agricultural drought refers
to situations in which moisture in the soil is no longer sufficient to
meet the needs of crop growing in an area due to insufficient
rainfall. Crops, particularly rain-fed crops, often have a minimum
overall threshold of cumulative rainfall for successful and healthy
plant development. For example sugar beet can consume up to 560 mm of
water during the growing season, depending on plant density, soils,
climate and weather conditions52. Crops, such as spring wheat, require
at least 350mm-400mm of rain for reliable yields53; for dry-land corn
farming 450-500mm or more is required for high yields during the
growing season54. These water requirements must be met by natural
rainfall, stored soil moisture from precipitation prior to the growing
season or from supplemental irrigation. A deficit of rainfall
therefore below these levels, in the absence of irrigation, can cause
plant moisture stress that affects development and consequently
reduces yields. A simple cumulative rainfall index could be developed
therefore to suit a grower’s specific requirements with regard to such
decreases in rainfall and therefore yield. By looking at historical
yield data, for example, an empirical relationship between seasonal
cumulative rainfall and yield can be established. However, the
distribution of rainfall during the growing season or at specific
stages of a plant’s development is often more important and customized
indices must be developed to capture this risk55. Such indices may
also include other weather parameters, such as temperature and
relative humidity.
A good index must account for the susceptibility of crops to moisture
stress during the different stages of development, taking into account
the biological and physiological characteristics of the crop and the
properties of the soil. Although actual soil moisture – the amount of
water present in the soil that is available for plant uptake – is not
in general an observed variable at meteorological weather stations,
given knowledge of the crop and soil type, rainfall and temperature
data can be used to create objective indicators to capture drought
risk during the various stages of a plant’s development. Crop growth
models or historical yield data can be used to infer the empirical
relationship between rainfall amounts and yield/quality for specific
soil and crop types.
For example, it is known that winter wheat yields can be strongly
influenced by plant moisture stress during the leaf-tube formation to
milking stages of development. Crop growth model results for the
territory of Kherson, Ukraine, show how wheat yields vary as a
function of deviations in cumulative rainfall and average air
temperature from normal levels during this period, assuming average
weather conditions for the rest of the growing season in the region
(Table 4)56:
Table 4: Deviations in Average Temperature and Cumulative Rainfall for
15 April - 14 June and their Impact on Winter Wheat Yields in Kherson
Deviation in Average Temperature Taverage from Normal (deg C)
Deviation in Cumulative Rainfall CR from Normal (%)
Decrease in Winter Wheat Yield at Harvest
0 < Taverage – Tnormal < 1
(CRnormal – CR)/CR < 50%
20%
1 < Taverage – Tnormal < 3
60% < (CRnormal – CR)/CR < 90%
20%
1 < Taverage – Tnormal < 3
50% < (CRnormal – CR)/CR < 60%
25%
1 < Taverage – Tnormal < 3
(CRnormal – CR)/CR < 50%
30%
Taverage – Tnormal > 3
60% < (CRnormal – CR)/CR < 90%
30%
Taverage – Tnormal > 3
50% < (CRnormal – CR)/CR < 60%
40%
Taverage – Tnormal > 3
40% < (CRnormal – CR)/CR < 50%
50%
Taverage – Tnormal > 3
(CRnormal – CR)/CR < 40%
100%
In Table 4, Tnormal is defined as the 10-year average value of Taverage
for Kherson weather station for the period 15 April – 14 June; CRnormal
is defined as the 30-year average CR value for Kherson weather station
for the period 15 April – 14 June. A grower could therefore protect
himself from moisture stress risk on his winter wheat crop by
purchasing a weather contract whose payout characteristics reflected
the expected yield losses estimated by the model results in Table 4.
General indices, such as the Palmer Drought Severity Index (PDSI)57,
have also become widely used drought assessment tools; the U.S.
federal government and many U.S. state governments rely on the PDSI to
trigger drought relief programs. The PDSI is based on more than just
rainfall and uses temperature, latitude, available water holding
capacity of the soil as well as precipitation to infer the supply and
demand of the soil moisture at a location on a weekly basis throughout
a growing season. The value of the PDSI is reflective of the how the
soil moisture, excess or deficit, compares with normal conditions.
Such an index could be used as a general indicator of the severity of
weather and therefore growing conditions for the local area, rather
than for a specific crop.
Excess Rainfall. Excessive moisture conditions, however, can also
retard growth and affect the yield and quality of a harvest. Excess
precipitation can cause flooding and water-logging of the soil, which
can restrict oxygen supply to root systems, reduce nutrient uptake,
lead to nitrate leaching and an increase in the incidence of plant
disease and pests58. The effects are worse when combined with warmer
than average temperatures which encourage pest development; warm water
also contains less dissolved oxygen than cold water. Precipitation can
impact the time and effectiveness of farming operations such as
sowing, land preparation and pesticide and fertilizer applications.
Excessive rainfall at harvest time can also delay harvest and/or spoil
standing crops. Daily rainfall amounts in excess of 4 mm59 can make
harvesting impossible – a grower could purchase weather protection for
such an event(s) that would cover the associated financial cost of a
harvest delay.
Quantifying the Risk
Once the index has been identified, it must be calibrated to capture
the financial impact of the specified weather exposure as measured by
the index. There can be two approaches to this stage: identifying the
financial exposure per unit of the defined index and/or establishing
the limit, the total financial protection required per risk period,
i.e. the maximum payout necessary in a worst-case scenario. The
approach that is chosen depends on the nature of the underlying index
and weather event. For example if the weather exposure is event
driven, such as a Category 5 hurricane hitting a particular location
or a cold winterkill event destroying an entire wheat crop, the latter
approach is more appropriate. If the weather exposure is of a
cumulative nature, such as drought or Growing Degree Days, the former
approach should be chosen. However, taking into consideration the
maximum protection required per risk period can also inform the
financial exposure per unit index.
Unit Exposure. Once weather indices to capture the impact of adverse
weather conditions on a specific crop’s yield have been developed it
is straightforward to calculate the financial impact of these events
for producers. In designing the index expert scientific
agro-meteorological assessments, either in conjunction with crop model
output or with verification using historical yields, have been
employed to construct an underlying index that best proxies the
weather sensitivity of the crop in question (e.g. Box 1). Having
identified the index, the crop yield, Y, or volume, V, variability per
unit of the defined index, I, can be defined i.e.
----------------------------------------------------------------------
Y = V / H = a(I) I (2)
where a(I) is some function of I that relates the index to the yield Y;
and H is the planting area of the crop. In order to calibrate an
appropriate weather contract the variation in crop yield must now be
converted into a financial equivalent that mirrors the producer’s
exposure. This can be done, for example, by considering a producer’s
production and input costs per hectare planted or by considering his
expected revenue from the sale of the crop at harvest. Producers with
fixed-price delivery contracts or those that use price risk management
instruments to protect themselves from market fluctuations in the
price of their crop at harvest time know the financial value of each
kilogram or metric tonne they produce and hence can quantify the
financial cost of a shortfall in production. For example, if a grain
producer knows he will receive $X per metric tonne of crop, the
following relationship must hold for his change in revenue:
-----------------------------------------------------------------------
Revenue = X * ( Actual Yield – Expected Yield ) * H = X * V = X * H
* a(I) * I
(3)
A good weather hedge must offset the negative Revenue fluctuation in
the event of a drop in yield from budgeted levels if a producer is to
protect his earnings. In order to perfectly replicate his position,
the farmer could enter into a weather contract with the following
incremental payout P per unit index
P = - X * H * a(I) * I (4)
Therefore his overall position would be:
Revenue + P = X * Y * H - X * H * a(I) * I = 0 (5)
Producers may have contractual obligations to deliver a pre-defined
amount of their farmed product to a buyer at harvest time, with
associated penalties if these obligations are not met. In such a
situation it would be straightforward to quantify and structure a
hedging product to protect a producer from these contractual costs in
the event of a weather-related shortfall in production (Box 2).
Often before a growing season, however, growers are uncertain as to
the price X at which they can sell their crop after harvest, rendering
the quantification of risk more uncertain. Furthermore, commodity
prices also often vary in response to extreme production shocks and it
is often difficult to quantify the production (weather)-price
correlation, particularly in emerging commodity markets where prices
are not always stable. However estimates for the harvest-time price
can be made e.g. the futures price for harvest delivery contracts
trading on a local commodities exchange, last year’s harvest-price or
the five-year average sales price could be used as a best estimate.
Furthermore historical sales price, marketing and production data can
be used to quantify the index-value interaction capturing the
financial exposure of a producer. By performing, for example, a linear
regression on historical commodity prices and farm production data, a
producer can quantify how a variation in index and therefore yield has
related to a variation in sales price of the commodity in the past to
estimate a value of X for the future. Such an approach can also be
used by an agrochemical producer to find the relationship between the
historical values of an index designed to capture the development of a
pest and the historical sales of pesticides, in order to quantify a
hedge to protect against reduced sales arising from weather conditions
that decrease pest development.
The Limit. Most weather contracts have a limit, which corresponds to
the maximum financial payout or recovery from the contract in a
worst-case scenario, such as a complete crop failure. The maximum
payout can be set by either considering the value-at-risk for the
producer in the event of a total crop failure or by looking at
historical index, production and sales data to find the worse-case
scenario historically in order to establish a limit. Alternatively a
producer may simply want to insure his production and input costs in
order to recover these outlays if the crop fails. If a producer’s
production costs are $Z per hectare farmed, $Z will therefore
correspond to the maximum payout, the limit of the weather contract,
for each hectare the producer wishes to insure. The unit exposure P
will therefore be:
P = ( - Y / Expected Yield ) * Z = ( - a(I) I / Expected Yield ) *
Z, for Y < 0 (6)
Structuring The Product
i. Structure Type
=================
Once the index has been identified and calibrated, the next step is to
structure a contract that pays when the specified adverse weather
occurs in order to perform a hedging or risk smoothing function for an
agricultural grower or producer. Derivative and insurance products
form the mainstay of the weather risk management market. While the two
instruments feature different regulatory, accounting, tax and legal
issues (see below, Section 2), the risk transfer characteristics and
benefits are often the same. One of the drivers of market growth has
been the flexibility between both instruments and the possibility to
tailor risk management solutions to a client’s needs60. A risk
management product can be:
*
A traditional insurance-style product – that is risk transfer that
results in downside protection in exchange for a premium, e.g. a
call or put option structure;
*
A risk-exchange derivative-based product – that is giving away
upside in good years or seasons to finance downside protection,
e.g. a collar or swap structure;
*
A risk-financing product to smooth risk over a longer time horizon
– that is using future income potential to guarantee the repayment
for an insurance payout in the event of a bad year, e.g. a finite
risk program.
Call and Put Options
A call option gives the buyer of the option the right, but not the
obligation, to buy the underlying index at a pre-defined level at the
maturity, or end date, of the contract61. In exchange for this right
the buyer pays a premium to the seller. Similarly a put option gives
the buyer the right, but not the obligation, to sell the underlying
index at a pre-defined level at contract maturity; in exchange for
this right the buyer of the option pays a premium to the seller. Every
option contract, and most weather contracts in general, are defined by
a set of standard specifications including:
1.
The reference index, I, and weather station(s) – complete
specification of the index and data used to construct it;
2.
The term, T – the risk protection period of the contract,
including the start and end date of the contract;
3.
A strike, K – also known as an attachment level, the level at
which the weather protection begins;
4.
The payout rate, X – the financial compensation per unit index
deviation above (call) or below (put) the strike at maturity,
defined as the unit exposure in the previous Section;
5.
The limit, M – the maximum payout per risk protection period.
The payout, Pcall, of a call option can be defined by the following
equation:
Pcall = min( max( 0, I – K )*X , M ) (7)
The payout, Pput, of a put option can be defined as:
Pput = min( max( 0, K - I )*X , M ) (8)
The type of option purchased depends on the risk profile of the buyer.
For example, assume a winter wheat grower loses 4% of his expected
yield every day the maximum daily temperature is above 30 degrees
Celsius in the months of May and June, incurring a cost of €16 per
hectare of wheat cultivated every day the 30 degrees Celsius threshold
is exceeded. The grower has 10,000 hectares of wheat under cultivation
and is prepared to accept yield loses due to heat stress of up to
€480,000, but wants protection for any loses in excess of that amount.
In this case, the grower may consider purchasing a call option, either
in derivative or insurance form, with the following specifications:
Reference Weather Station (RWS): Growerstown, ID No. 12345
Index: Daily Tmax > 30 C, measured at RWS
Calculation Period: 1st May 2005 – 30th June 2005 (inclusive)
Call Strike: 3 events
Payout Rate: €160,000 per event above the strike
Limit: €1,600,000
For securing such protection the grower is required to pay a premium,
but it allows the grower to recover €160,000 for each day in May and
June that the daily maximum temperature exceeds 30 degrees Celsius in
excess of the strike level. Figure 5 illustrates the impact of such
hedging strategy on the revenues of the grower – his downside exposure
is now limited to €480,000 by purchasing the call option, unless the
number of heat events exceeds an unprecedented 13 during the
calculation period. Modifications can obviously made to this
simplified example to better replicate the exposure of the grower – a
more sophisticated product may be, for instance, based on an index
that considers only consecutive days of excessive temperature,
includes relative humidity or a non-linear payout rate that increases
the compensation as the number of heat events during the calculation
period increases. Alternatively the grower many want to purchase a
digital call option, an all-or-nothing structure, that will pay the
grower a lump sum, rather than incremental payout, if the heat stress
reaches a critical level at which most of the crop will be lost. An
example of a put option application is given in Box 3.
Collar
A business may be averse to paying an upfront premium for risk
protection. An alternative it can consider is to enter into a contract
where the business receives downside protection in return for
sacrificing upside revenue in the event of weather that is beneficial
for the business. In essence the business can forego a proportion of
profit to offset the cost of reduced revenues. It can do this by
selling a put option and then buying a call option from the provider,
or vice versa. A collar therefore combines both a call and put option,
but does not involve an exchange of premium from the end user to the
provider. Rather a collar is a means by which two parties can exchange
risk, hence collars may often be structured with asymmetric call and
put options in order to make the risk exchange of equal value to both
parties. This approach may not be applicable to all weather risk
management problems in agriculture. Furthermore, businesses may be
averse to giving up profits in a good year. However, a very simple
example of a possible application can be found by considering a local
agrochemical company whose sales of a particular pesticide vary
depending on the number of pest growing degree days (PGDDs) recorded
in their sales region during the winter. When the recorded PGDDs are
high, pest attack incidents increase and pesticide sales increase
accordingly. When PGDDs are low demand for their product drops and
pesticide sales are low. The company has quantified this risk and
finds that on average it loses or gains $12,000 per PGDD from their
budgeted revenues, if the accumulated PGDD are below or above the 1700
PGDDs expected in a normal winter the region. The company may be
interested in a collar, as not only is it costless to enter into a
collar agreement, but it also reduces the company’s revenue volatility
caused by weather. In this case, the company may consider purchasing a
collar with the following specifications:
Reference Weather Station (RWS): Growerstown, ID No. 12345
Index: Cumulative PGDDs measured at RWS
Calculation Period: 1st November 2005 – 31st March 2006 (inc)
Call Strike: 1800 PGDDs
Put Strike: 1600 PGDDs
Payout Rate: $12,000 per PGDD above/below strikes
Limit: $2,400,000
The historical distribution of November-March PGDDs in Growerstown is
found to be symmetric around the 1700 PGDD average with a standard
deviation of 100 PGDDs, hence the call and put options have strikes
equidistant of the average to create a zero-cost collar. Figure 6
illustrates the impact of such hedging strategy on the revenues of the
company – the collar reduces a potential two standard deviation
fluctuation in revenues for the company from +/- $2,400,000 to +/-
$1,200,000.
Swap
A swap is a contract in which a buyer makes a payment to the seller
when a weather index rises above a pre-defined strike level and
entitles the buyer to receive a payment from the seller when the index
falls below the same level. Essentially a swap is a put and a call
option with the same strike, payment rate and limit, which, like a
collar, is costless to enter. In the example above, instead of using a
collar contract the local agrochemical company could “sell” a swap
contract to a provider with a strike of 1700 PGDDs and a payout rate
of $12,000 per PGDD. This would ensure that the business achieves no
more or less than its budgeted revenue. Swaps are derivative
over-the-counter (OTC) contracts that are commonly traded in the
secondary derivative weather risk market and, outside the energy
industry, are not often used by end users as they do not always offer
the best correlation to the underlying risk. Swaps are only available
in derivative form62.
Exotic Structures
In theory a weather risk management solution can take any form or
combination of options, swaps, triggers and indices. Possible exotic
combinations include: knock-in or knock-out options, which grant the
buyer a standard call or put option if a particular knock-in or
knock-out threshold is breached, either on the same or even a
different index – for example a heat stress call option for wheat,
that is only triggered if precipitation during the same calculation
period drops below a critical level; compound options, known as “an
option on an option”, that grants the buyer to right to purchase an
underlying option at some future date – for example a multi-year
structure that gives the buyer an option to buy an option on the
weather conditions for the next growing season at the end of the
current season; structures with a variable start date depending on the
timing of a pre-specified event – such a structure may be appropriate
for crops with variable planting dates that can be associated with
cumulative rainfall or growing degree day totals.
Reference indices may also include non-weather variables. For example,
temperature contingent commodity call options that give a purchaser
the right but not an obligation to buy an underlying commodity as a
pre-specified price and volume only if certain temperature, i.e.
growing conditions, have been met. Such exotic structures could
potentially provide total revenue insurance for agricultural producers
whose revenues depend on both the price at which they sell their
produce and the volume that they produce. Such contracts exist and are
traded in the OTC energy derivative markets.
Finite Risk Solutions
Finite risk products are becoming another accepted risk management
mechanism, often popular with corporate end users. Finite risk
solutions seek to spread the risks for an insurance policyholder over
time and shift the main value proposition from traditional risk
transfer towards risk financing for the client. Products are designed
specifically to the needs of the customers, and in contrast to
traditional annual-renewal insurance policies, they are typically
multi-year contracts that smooth the year-to-year volatility of
insurance claims and premium payments – and consequently earnings –
over a long time horizon therefore limiting the overall risk transfer
during the contract period. Finite risk programs involve either
pre-funded (prospective) structures – where the client pays an annual
or single premiums into a fund account that earns a contractually
agreed investment return; these funds are then used to make loss
payments to the customer – or post-funded (retrospective) structures,
where the client pays back the claims payments of the insurer over a
defined period of time. Such a risk management mechanism can also be
used to finance weather risk.
Exchange Traded Products
The Chicago Mercantile Exchange (CME) lists weather futures and
options on futures, with over $1.6 billion dollars of trading notional
value in 200363. These are standardized exchange-traded derivatives
reflect monthly and seasonal temperatures of 15 U.S. cities, five
European cities and two Japanese cities, measured according to
specific indices and weather stations. The contracts are specifically
tailored for the energy industry. Weather contracts for winter months
are classified according to an index of cumulative Heating Degree Day
(HDD)64 values, days in which energy is used for heating. Weather
contracts for summer months in the U.S. are classified according to an
index of cumulative Cooling Degree Day (CDD)65 values, days in which
energy is used for air conditioning, and in Europe according to an
index of cumulative average temperature. All futures and option
contracts on the CME can be bought and sold through an exchange
broker, with terms and conditions set forth in agreements provided by
the CME. However, due to the standardized nature of the contract they
may have limited appeal to the agricultural industry.
ii. Risk Retention and Premium
It is clear that an important aspect to consider when structuring an
index-based solution is the retention of risk by the party seeking
protection, this means defining the index trigger level where the
weather protection begins. The strike determines the level of risk
retention of the insured party and is the key to pricing and success
in transferring the risk. A strike very close to the mean of the index
indicates a low level of risk retention by the end user and a contract
that will pay out with high probability. This implicitly means a large
premium, as well as the possibility of inspiring little interest from
the weather market if the location or nature of the risk is outside
the main liquid trading hubs or variables. A strike further away from
the mean reduces the probability of a payout and hence the premium of
the contract as the entity is retaining the more frequent,
near-the-mean, risk internally and transferring less to the market.
The level of risk retention will depend on the risk appetite and
business imperatives of the end user in question and its sensitivity
to the premium associated with entering into a contract. For instance,
to reduce the premium payment the wheat grower in the call option
example above could increase the strike for heat stress events. By
retaining more risk, all things being equal, it would reduce the
premium of the contract. Alternatively the grower could reduce the
payment rate to partially, instead of fully, hedge its exposure.
Premium payment terms must be defined before entering a weather
contract and an overview of how such contracts are priced by weather
market providers is given in the following Section.
Execution
=========
i.
The Market Providers
The main providers of risk capacity, product structuring and/or
pricing for end user customers in the current weather risk market can
be categorized into three main groups:
*
Insurance and reinsurance companies, who see non-catastrophic
weather insurance as a natural extension to their traditional
business and given analysis capabilities. Examples include: ACE,
AXA, Munich Re, Partner Re, Swiss Re, Tokio Marine and Fire
Insurance, XL Capital. Most of these entities can also offer
derivative products and, although some many choose to retain the
risk by dealing in a large amount of diversified end user
business, several are some of the most active portfolio managers
in the secondary market, using financial derivatives contracts
to manage their weather risk portfolios, of both high and low
frequency risk;
*
Banks, who structure weather risk solutions to fit the needs of
their clients. Examples include: ABN AMRO, Calyon, Deutsche
Bank, Goldman Sachs, Merrill Lynch, Rabo Bank. Banks have a
large potential client base for weather derivative products and
may find many marketing and cross-selling opportunities in many
different sectors of business. Banks generally do not have as
much risk capacity as the (re)insurers, often passing positions
of their end user customers to other market providers or
actively hedging positions in the secondary OTC and
exchange-traded derivatives market;
*
Specialized hybrid companies or funds such as Coriolis Capital
(formerly Société Générale) and Guaranteed Weather Trading Ltd.,
have been established specifically to trade and invest in
weather risk. Such hybrid entities are able to deal in weather
derivatives and reinsurance and offer weather risk solution
products to customers;
Brokers, who facilitate transactions between buyers and sellers of
weather protection, are an independent point of contact for customers
seeking a counterparty in the weather market. A broker’s role is to
allow for price discovery while ensuring confidentiality until final
negotiations are concluded. Brokers have in-depth knowledge of the
market with some offering risk solution structuring services.
Derivative brokers, dealing primarily with the secondary OTC and
exchange-traded market include: Tradition Financial Services (TFS),
Intercapital (ICAP), GFI and Evolution. Insurance brokers, that
provide weather insurance broking services, include Marsh, Aon and
Willis Group.
The energy companies responsible for the birth of the marketplace,
Enron, Aquila, Southern Company and Entergy Koch (now Merrill Lynch)
are no longer active in the weather market. Although the market is
still predominantly driven by energy related weather risk – with
energy companies and several bank’s hedging their energy portfolios
with weather derivatives – the major source of secondary market
liquidity is now driven by the three predominant types of
counter-party outlined above, either through the hedging of end user
deals or taking speculative positions.
ii. Regulatory Issues66
Depending on the jurisdiction, weather risk management products can be
classified as either financial (derivative), insurance or gaming
contracts. Depending on their classification, these contracts are
subject to specific tax and accounting treatments, which can render
one form more optimal than another for an end user’s purposes and
business. Interested parties are strongly advised to contact their
local financial services authority, insurance regulator or a
professional specializing in insurance law to find out how weather
contracts are treated in their jurisdiction and the legal and
financial implications associated with each.
In Europe, individual countries currently have their own regulatory
standpoint. In the U.K., for example, OTC weather derivatives are
considered to be investment or financial contracts, “contracts for
differences”, and are therefore regulated by the U.K. Financial
Services Authority along with other financial contracts. Entities
trading in weather derivatives must either be licensed or authorized
to trade derivative contracts or be exempt from obtaining a license,
such as end users or those dealing in weather mitigation products on
their own account67. The ISDA Master Agreement68 confirmations and
credit support provisions are used for the documentation for OTC
weather derivatives in the U.K. and various countries in Continental
Europe have also adopted this documentation69. Germany, France and The
Netherlands have a similar regulatory approach as the U.K.; in other
European countries the classification of such contracts has not yet
been clarified, e.g. Italy.
However, the new EU Investment Services Directive (ISD2) on financial
instruments markets may bring weather derivatives into the regulatory
framework that applies to other derivative contracts and give a
EU-wide “passport” for trading OTC weather derivatives70. Member
states have until early 2007 to bring their national laws and
regulations into line with the new rules. However, they will not be
able to finalize their national implementation programmes until the
European Commission conclude their discussions, including if weather
derivatives should be treated as financial instruments subject to the
Directive or as an asset class outside the scope of regulation. If the
Directive is extended to cover weather derivatives, then member states
will be required to adjust and apply their licensing and other
financial regulatory rules accordingly. The proposal to extend the
scope of regulation to cover weather derivatives will have several
benefits and disadvantages for market participants71, however there
are some exemptions included in the Directive. In particular, for
market players that currently can operate without a financial services
license, such as end users of weather derivatives products.
In the U.S., OTC weather derivatives are also treated as financial
contracts, and most market participants use an ISDA Master Agreement
to document their weather derivative transactions, although weather
derivatives are excluded from the regulatory scope of the Commodity
Exchange Act72; exchange-traded contracts are regulated by the
Commodity Futures Trading Commission73.
If a company wishes to purchase weather insurance to mitigate its
weather risk, it must do so from an insurance provider licensed in its
jurisdiction, who in turn must draft an insurance policy that must
meet the definitions of “insurance” in the jurisdiction where the
policy is to be written. In comparison to financial contracts,
insurance is highly regulated and must comply with strict criteria.
Regulators define the elements of an insurance policy to ensure
supervision and compliance with the law. While definitions vary from
jurisdiction to jurisdiction, the following six common elements can be
extracted74:
1.
The process of insurance involves a contract between two parties,
which runs for a specified term of “cover”.
2.
Under the terms of this contract, one party (the “insurer”) must
promise to pay a sum of money or provide a corresponding benefit
to the other (the “insured”), should a specified future contingent
event occur during the term of cover of the contract.
3.
The insured must make a payment of money or money’s worth – the
premium, in a lump sum or installments – to the insurer in
consideration of the insurer’s promise of the point above.
4.
There must be uncertainty as to the occurrence or the timing of
the specified future contingent event.
5.
There must be an “insurable interest” for the insured party. In
other words, the insured must have an economic interest in the
subject matter of the contract and have an interest in the subject
matter that is lawful.
6.
The insured must suffer a loss of a financial nature in relation
to his insurable interest and the amount payable must be a
reasonable recovery for the loss experienced.
To further illustrate point 6, the Weather Risk Management Association
writes75,
“In many cases the amount payable by the [insurer] may be quantified
by the actual financial loss suffered by the insured if the specified
uncertain future event occurs and causes damage to the subject matter
of the contract; this is in the nature of an indemnity. However, the
amount payable by the [insurer] may also be predetermined and may be
more or less than the loss suffered by the insured. That said, these
“valued” policies or those that have fixed or formulaic payments are
employed in order to expedite the claim settlement process. Whilst
they do not provide a full correlation between the loss suffered by
the insured and the amount paid by the insurer, the sums are not
entirely unconnected. In these contracts, the amount payable upon a
contingency must represent a genuine attempt to pre-estimate, or
reflect, the loss that the insured might be expected to suffer, should
the contingency occur. The amount payable cannot be wildly divergent
from the loss experienced. The essential feature of this element of
insurance remains that the insured does suffer a loss.”
Although a weather derivative is consistent with point 1 and may share
– although does not have to – some similarities with points 2-4 above,
such an instrument does not need by law to exhibit any elements of
insurance outlined by points 5 and 6. For example, the New York State
Insurance Department based its conclusion that a weather derivative is
not insurance by noting, "the issuer is obligated to pay the purchaser
whether or not that purchaser suffers a loss. Neither the amount of
the payment nor the trigger itself in the weather derivative bears a
relationship to the purchaser's loss. Absent such obligations, the
instrument is not an insurance contract.”76
In the context of agriculture, the elements of weather insurance are
therefore that the insured party must have an insurable interest,
needs to suffer a loss in respect of that interest and the insurance
payment must indemnify the insured party for the loss. For example, if
a grower wants to purchase weather insurance for his wheat crop, he
must: a) be growing wheat; b) purchase an insurance contract based on
an index that correlates closely to his wheat yield; and c) purchase
insurance whose payout is related to the actual financial loss
experienced. For instance the grower cannot buy insurance for more
hectares of wheat than the number of hectares he is currently farming,
nor can he purchase a contract where the sum insured (the limit) or
the payout rate exceeds a reasonable value for the crop – for example,
he cannot buy insurance with a limit of $10,000 per hectare when a
reasonable estimate of the value of the crop, under current market
prices or contractual obligations, is $1,000 per hectare. An end user
and the insurer must be clear that the contract into which they enter
satisfies the elements of insurance outlined by their jurisdiction,
either by conducting a correlation analysis between the end user’s
economics and variations in the weather in the application process
and/or requiring the insured party to represent and warrant in the
claim form that the recovery is a reasonable estimate of economic
loss. In jurisdictions where weather risk protection can be obtained
in both insurance and derivative form, such as in the U.S. or the
U.K., these contracts are subject to distinct tax and accounting
treatments.
Box 1: The Corn Grower’s Weather Hedge
======================================
A corn grower is worried about his corn production. He has a 3700-acre
dry-land farm and is worried that the growing degree accumulation for
the coming growing season will not be enough to ensure a good harvest.
The grower is one of the few corn producers in the region and he has
just won a contract to deliver 500,000 bushels of corn to a local
buyer at harvest. However there are penalties in the contract
associated with under-delivery. The grower remembers 2000 was a cool
summer when his farm yields were low and he is worried that this
summer will be the same. He has read about weather derivatives and is
interested to see if a weather derivative contract could adequately
protect him against this risk.
The grower grows a corn hybrid that needs 2500 GDDs to mature and
assure a maximum yield and quality harvest. He knows from the seed
company where he purchases the seed that his particular hybrid’s
maturity is rated using modified growing degree days (MGDDs) defined
as:
Daily MGDD = max(0, [ (max(0, 30 - Tmax) + max(0, Tmin - 10) ] / 2 –
10 )
where Tmax and Tmin are the daily maximum and minimum temperature
measured in degree Celsius for each day during the growing season. The
grower has a weather station on his farm, which he installed a year
ago, but he knows there is an official National Meteorological Service
(NMS) weather station in the local airport 10km away. The grower
purchases 30 years of daily maximum and minimum temperatures from the
local NMS office. He compares the past year of data from the NWS
station with the data he has been collecting on his farm for the past
year and he finds that the daily minimum and maximum temperatures
correlate highly with a correlation coefficient of 98% and an average
difference in temperatures of 0.3 deg Celsius. He is happy that the
NWS represents the weather on his farm well. The grower has seven
years of historical corn yield data from his farm. He wants to analyze
this data further, so he contacts the local Agricultural University in
a nearby town to asks if they would be willing to give him some
technical assistance and analysis advice. A postgraduate student who
is studying arable farming is interested in the project and agrees to
help.
The grower has always grown the same hybrid. He knows from experience
that the optimal planting date for corn in his region is May 6th, that
the hybrid corn he grows takes approximately 110-130 days to mature
and that he usually harvests his crop in the first week of September.
He knows during the later half of September and early October the
probability of frost in his region begins to increases, therefore he
knows he always wants to harvest before October. Using this
information the postgraduate calculates the cumulative MGDDs, as
defined by the equation the grower has from the seed company, for May
6th – September 15th, for the past seven years from the NMS data and
compares them to the historical yield data for the farm. He makes a
table and plots the results.
Table A: Corn Grower’s Historical Farm Yields versus Cumulative MGDDs
Year
Farm Yield (bu/acre)
MGDDs
(May 6th – September 15th)
1998
106.6
2551
1999
184.2
2651
2000
58.9
2249
2001
206.6
2602
2002
128.3
2399
2003
234.8
2649
2004
194.9
2550
He finds that there is a strong relationship between the accumulated
MGDDs at the local NMS station and his farm yield. The correlation
coefficient, r, between the interannual variations in MGDDs and the
interannual variations in yield is 85%, i.e. the variation in the
MGDDs explains nearly 73% of the total interannual variability of corn
yield on the grower’s farm. From the plot (Figure A) he can see that
there is a linear relationship between cumulative MGDDs and yield. He
performs a least squares regression on the data to find the best-fit
line, given by:
Corn Yield (bu/acre) = 0.365*MGDD – 761.1 (a)
---------------------------------------------
with a r2 of 72.7%. He can see that that linear relationship captures
the grower’s worst year, 2000, when the accumulated MGDDs were 2249
and his average yield was only 58.9 bu/acre. However he notices that
fit is not perfect – 1998 is an outlying year. The accumulated MGDDs
in 1998 were 2551, as in 2004, but his yield was nearly 50% less. The
postgraduate asks the grower about this. The grower tells the
postgraduate that 1998 was the first year in which his farm was in
operation. Normally rainfall in his region is plentiful for dry-land
farming of corn, with over 500 mm of rainfall on average during the
growing season. However he remembers 1998 was an extremely dry year
and, being a new grower, he knew that in that particular year he
hadn’t appropriately reduced his seeding and fertilizer rates to
accommodate the low levels of soil moisture in his fields prior to the
growing season. However he knows that this year the stored soil
moisture on his land is adequate to for good crop development. Using
this new information the postgraduate decides to remove 1998 from his
regression and finds the new relationship between corn yield and
cumulative MGDDs to be:
Corn Yield (bu/hct) = 0.38*MGDD – 791.8 (b)
-------------------------------------------
with a r2 of 91.3%. The postgraduate is now confident that MDGGs are a
good index for corn production on the grower’s farm.
Figure A: Historical Modified Growing Degree Days vs. Grower’s Corn
Yield
Box 2: The Corn Grower’s Weather Hedge
The grower is one of the few corn producers in the region and he has
just won a contract to deliver 500,000 bushels of corn to a local
buyer at harvest. However there are penalties in the contract
associated with under-delivery. In the contract the buyer has
specified that the grower will receive $3 per bushel for the 500,000
bushels he is contracted to deliver, however the contract also
stipulates that the grower will pay a penalty of $1 for every bushel
under 500,000 that he fails to deliver. The grower is extremely happy
with his contract. He knows on can average he can expect to make $2.5
per bushel for his production by selling outside of his area, so $3
per bushel is a very good price for the grower as it does not include
transportation costs. Additionally he also knows that he can sell his
excess production, if any, at $2.5 per bushel. However he is concerned
about the $1 per bushel penalty so he makes a table of his overall
revenue with and without the supply contract given different farm
production scenarios.
Table B: Farm Revenue under Different Total Production Scenarios
Total Farm Production (bushels)
Revenue with 500,000 bu Supply Contract ($)
Revenue without 500,000 bu Supply Contract ($)
800,000
2,250,000
2,000,000
700,000
2,000,000
1,750,000
600,000
1,750,000
1,500,000
500,000
1,500,000
1,250,000
400,000
900,000
1,000,000
300,000
550,000
750,000
200,000
200,000
500,000
He is interested in purchasing a weather contract that duplicates the
terms of his underlying corn supply contract so that in years where
his production falls below the 500,000 bushel threshold he does not
make less money than he would expect to make if he simply sold his
produce outside of the region at $2.5 per bushel.
He can see that every 100,000 bushels below the 500,000 bushel
threshold corresponds to a loss in revenue of $100,000. The grower
would like to enter a contract that financially compensates him for
such a loss in case there is a weather-related shortfall in production
on his farm. The grower uses the postgraduate’s equation (b) to
convert the total farm production in bushels to an equivalent
cumulative MGDD:
MGDD = (Total Production / 3700 + 791.8)/0.38 (c)
-------------------------------------------------
He finds that 2436.5 MGDDs corresponds to 500,000 bushels of farm
production according to the equation. For total farm production less
than 500,000 he makes a net loss in revenue of $1 for each bushel less
than the 500,000 bushel threshold, i.e.
Δ MGDD = ( $1 / 3700 ) / 0.38 = 0.00071 (d)
-------------------------------------------
Therefore for every MGDD less than 2436.5 MGDDs from the May 6th –
September 15th he calculates that he can expect to lose $1,410 per
MGDD in revenue.
Table C: Total Farm Production and Required MGDDs
Total Farm Production (bushels)
Required MGDDs
Loss in Expected Revenue Associated with Entering Supply Contract ($)
800,000
2649.3
0
700,000
2578.4
0
600,000
2507.4
0
500,000
2436.5
0
400,000
2365.6
-100,000
300,000
2294.7
-200,000
200,000
2223.8
-300,000
100,000
2152.8
-400,000
Box 3: The Corn Grower’s Weather Hedge
Having quantified his financial exposure as $1,410 per MGDD in the
event of a cool summer, the corn grower decides that he should
purchase a weather derivative contract that pays out when MGDDs
accumulated at his local National Meteorological Service (NMS) weather
station at Corntown Airport are low. In particular, given his
analysis, he wants to protection against years where the cumulative
MGDDs, from May 6th – September 15th, are less than 2436. He decides a
put-option structure is the best way manage this risk and he develops
a prototype weather contract with the following specifications:
Reference Weather Station (RWS): Corntown, ID No. 56789
Index: Cumulative MGDDs
Calculation Period: 6th May 2005 – 15th September 2005 (inc)
Put Strike: 2436
Payout Rate: $1,410 per MGDD below the strike
Limit: $400,000
The grower contacts his local derivatives broker to see whether the
broker can get him a quote for the prototype contract he has
developed.
3. Valuing Weather Risk
=======================
Pricing Overview
The premium of an index-based weather contract is determined
actuarially, that is by conducting a rigorous analysis of the
historical weather in order to understand the statistical properties
and distribution of the defined weather index and therefore the
payouts of the insurance or derivative contract. Such an analysis
includes: a) cleaning and quality controlling the data, i.e. using
statistical methods to in-fill missing data and/or to account for
significant changes, if any, as a result of instrumentation or station
location changes; b) checking the cleaned data for significant trends
and detrending to current levels if appropriate – this is particularly
pertinent for temperature data which, in general, exhibits a strong
warming trend in the Northern Hemisphere and; c) performing a
statistical analysis on the cleaned and detrended data and/or a Monte
Carlo simulation, using a model calibrated by the data, to determine
the distribution of the defined weather index and therefore payouts of
the contract. By determining the frequency and severity of weather
events specified by the index an appropriate premium can be
calculated.
It should be noted that the premium charged by the providers in the
weather market may depend on several factors, not all as objective as
the underlying statistical analysis of the weather data. Institutions
charge different risk margins, or discounts, over the expected value
or fair price to potential buyers; these choices are driven by the
risk appetite, business imperatives and operational costs of the
provider77. An overview of pricing is given in this Section and the
implications of the premium charged for the end user will also be
discussed. The data issues associated with points a) and b) will be
covered in Section 4.
i.
Expected Loss and Risk Margin
To illustrate the pricing process let us consider an index-based
weather contract structured as a call option (Section 2). The payout,
P, of the contract is determined by the following equation:
P = min( max( 0, I – K )*X , M ) (9)
where K is the strike, I is the index measured during the calculation
period, X is the payout rate per unit index and M is the limit of the
contract. To calculate the premium for the contract one must determine
the following parameters:
1.
The expected loss of the contract, E(P), i.e. the average or
expected payout of the structure each year;
2.
The standard deviation of the payouts of the contract, (P), i.e.
a measure of the variability of the contract payouts;
3.
The xth-percentile of the payouts, i.e. a measure of the
value-at-risk (VaR) of the contract for the seller, VaRX(P). For
example, the 99% VaR represents the economic loss for the provider
that is expected to be exceeded with 1% probability at the end of
the calculation period of the contract.
These three parameters quantify the expected (1) and variable or risky
(2, 3) payouts of the contract and must be determined from the
historical weather data, either by using the historical index values
from the available cleaned and detrended dataset or by using the data
to calibrate a Monte Carlo simulation model to generate thousands of
possible realizations of I in order to fill-out the distribution of
payouts and to determine better estimates of E(P), (P) and VaR99(P).
A complete description of the various methods for determining these
payout statistics are beyond the scope of this Chapter, but an
overview of possible approaches is given in the following subsection.
It is clear however that E(P), (P) and VaR99(P) will vary with the
strike, payout rate and limit.
Having established values for the expected and variable payout
parameters, the price of a contract is then determined by the risk
preferences of the (re)insurance company or financial institution that
is providing the risk protection: that is, how they measure the cost
of risk with respect to return for the purposes of pricing, risk
management and capital allocation78. As a result this is the most
subjective aspect to the risk pricing process as it is largely driven
by the institutional constraints and risk appetite of the provider.
However it is clear that the provider will charge E(P) plus an
additional risk margin for taking the weather risk from the end user,
i.e.
Premium = E(P) + Risk Margin (10)
where E(P) is the expected loss of the contract for the provider which
can be mathematically expressed as:
(11)
where n is the number of simulation trials or historical values of I
used to compute the expected loss. The risk margin can be further
broken down to include the following components79:
Risk Margin = CL + EL ± CLD (12)
--------------------------------
CL is the capital loading which is related to the cost of risk capital
needed by the provider to underwrite or sell a particular weather
index contract. This loading usually includes the profit margin and is
typically the predominant component of the risk margin, estimated as a
function of the variability of the contract payout as measured by (P)
and/or VaRX(P). EL is the administrative expense loading, which may
include internal management expenses and taxes and/or external
expenses for items such as brokerage and premium taxes. CLD represents
the commercial loading and discounts. Whereas E(P), CL and EL are
considered the technical premium, which in theory corresponds to the
price that allows the provider to achieve a target return on the risk
capital, CLD depends on the weather market conditions, the current
portfolio position of the provider and the overall relationship with
the client. For simplicity we will refer henceforth to the variation
in premium from the expected loss as the risk margin.
There are many methods for measuring risk and hence determining the
risk margin of a risk taker. Two examples of simple methods that have
been suggested80 for the weather market include the Sharpe Ratio and
the Return on VaR methods – both measure expected excess return in
terms of some measure of risk and hence determine the “cost of risk”
for the contract seller:
*
Sharpe Ratio, = [ Premium - E(P) ] / (P)
*
Premium = E(P) + (P) (13)
*
Return on VaR (99%), = [ Premium - E(P) ] / [ VaR99(P) – E(P) ]
*
Premium = E(P) + [ VaR99(P) – E(P) ] (14)
The Sharpe Ratio uses standard deviation as the underlying measure of
risk and therefore represents the “cost of standard deviation” as
determined by the seller’s risk preferences. One of the benefits
relating risk with the standard deviation of payouts is that it is an
easy parameter to estimate, however it is a symmetric measure of risk
capturing the mean width of the payout distribution and for
traditional risk exchange products the payout distribution is often
not symmetric but has a long tail. The Return on VaR method uses
VaR(99%) as the underlying measure of risk and therefore represents
the “cost of VaR”. Value-at-Risk (VaR) is a term that has become
widely used by insurers, corporate treasurers and financial
institutions to summarize the total risk of portfolios. Central bank
regulators, for example, use VaR in determining the capital a bank is
required to reflect the market risks it is bearing. A VaR calculation
is aimed at determining the loss that will not be exceeded as some
specified confidence, often set at the 99% confidence level, over a
given time horizon.81 In the context of a weather risk management
product the natural time horizon to choose is the expiry date of the
contract. The advantage of VaR99 is that it is computed from the loss
side of the payout distribution, where loss is defined with respect to
the expected payout E(P), and therefore captures the potential
financial loss to the seller. Using the Return on VaR method is more
appropriate for pricing structures that protect against
low-frequency/high severity risk, that have highly asymmetric payout
distributions. However VaR99 is a harder parameter to estimate,
particularly for strike levels set far away from the mean, and is
usually established through Monte Carlo simulation. The worst-case
recorded historically can often be used as a cross check for VaR. In
both methods outlined above, and quantify the risk loading, given
by Equation 12, appropriate for the risk preferences of the provider.
It is also worth noting that weather market participants can often
enter into financial derivatives contracts to manage their weather
risk portfolios and actively hedge positions in the secondary OTC and
exchange-traded derivatives market. This is particularly true if the
end user risk is in a location that is included or positively
correlated to the locations that are commonly traded in the market.
Moreover, even if a market provider chooses to retain the risk
internally, a new potential contract may look attractive in comparison
to the overall portfolio of the risk-taker, i.e. it may be a contract
that, like hedging, will reduce the relative and VaR99 parameters
and hence the overall risk position of the portfolio. The cost of the
chosen hedging strategy (if any) may affect the expected loss and the
variability of payouts – as Robert Henderson82 notes, making the
payout a function of the hedging strategy H, P(H) (see Market Pricing
below) – hence a more general representation of the premium, given
Equations 10 and 12, is83:
Premium = E(P(H)) + ( (P(H), C) - (C) ) (15)
where (P(H), C) can be expressed as84:
(16)
(P(H)) and (C) are the standard deviations of the contract payout
and the current portfolio position C over the same time horizon
respectively; (P(H),C) is the overall standard deviation of the new
portfolio with the contract included; ρ(P(H),C) is the correlation
between the contract payouts and the position of the current portfolio
over the time horizon of the contract. As the correlation can be
negative or positive the new contract can decrease or increase the
overall risk of the portfolio, as can the relative magnitudes of (P(H))
and (C), and hence reduce or increase the premium while maintaining
the same cost of risk, . Alternatively the premium can be expressed
as:
Premium = E(P(H)) + ( VaR99(P(H), C) - VaR99(C) - E(P(H)) ) (17)
where VaR99(P(H)) and VaR99(C) are the VaR(99%) for the contract
payout and the current portfolio position C over the same time horizon
respectively, and VaR99(P(H),C) is the VaR(99%) of the new portfolio
with the contract included. As VaR(99%) may express the 99% confidence
level of a non-normal distribution of payouts, it is difficult to
express VaR99(P(H),C) mathematically, estimates are usually taken from
simulation results. A well-diversified book of contracts will reduce
the relative and VaR99 parameters and hence the of the overall risk
position of the portfolio of a provider.
A reasonable estimate for , given prices in the weather market are
= 15-30% and = 5-10%.
ii. Approaches to Pricing Weather Risk
In order to price a weather contract given the overview above, the
parameters that quantify the expected (E(P)) and variable (VaR99(P), (P))
payouts of the contract must be determined. This section briefly
outlines four possible approaches, with varying degrees of difficulty
and effort, that are commonly used by weather market participants; the
first three methods are based on analysis of historical weather data
and the fourth is based on prevailing market price of weather risk. In
general, providers may use several or all of these methods to
crosscheck results and compute a contract price.
Historical Burn Analysis
Historical Burn Analysis (HBA) is the simplest method of weather
contract pricing. It involves taking historical values of the index,
which may be based on raw, cleaned and possibly detrended weather
data, and applying the contract in question to them. Assuming the data
used to calculate the historical indices is of good quality for the
risk analysis, HBA can give a useful and intuitive first indication of
the mean and range of possible payouts of a weather contract from
which parameters such as E(P) and (P) can be calculated (Box 4). The
method is simple and can be easily done in a spreadsheet. The
disadvantage of HBA is that it gives a limited view of possible index
outcomes: it may not capture the possible extremes and may be overly
influenced by individual years in the historical dataset. Estimates of
parameters such as VaR99(P) therefore become very difficult, although
the largest historical value is always a good reality check when
considering the possible variability of payouts. Additionally the
confidence level that can be attached to averages and standard
deviation calculated using historical data is limited by the number of
years of data available. The standard error in the average decreases
as the number of years included in the average increases however,
although weather stations often have 30-40 years of historical data –
the representative nature of older data for today’s weather and
climate should also be questioned (Section 4).
Historical Distribution Analysis
Much can be gained in understanding the statistical properties of the
underlying index. If index values are calculated from historical
meteorological data, then looking at the distribution of these index
values and ascertaining the probability distribution function of the
index can give a better estimate of the parameters necessary to
specify that function and therefore the expected and variable payouts
of the contract. Historical Distribution Analysis (HDA) involves
determining the probability distribution that best fits the historical
(possibly detrended) index data. The process is very much one of trial
and error and various standard tests and goodness-of-fit statistics,
each with their strengths and weaknesses, can be used to pick the best
distribution from a potential selection, such as Quantile-Quantile
plots, calculation of moments and statistical tests such as
chi-squared, Kolmgorov-Smirnov, Anderson-Darling, root-mean squared
error and maximum likelihood methods85. By determining the
distribution and therefore the parameters necessary to define it, such
as the mean and standard deviation, the E(P) and (P) VaR99(P) can be
calculated either by simulation from the distribution (see below) or
analytically, depending on the type of distribution chosen and the
underlying complexity of the contract to be priced. For example in the
case of a normally distributed index, closed-form expressions can be
found for E(P), (P) and VaR99(P) for simple structures such as call
and put options86,87. For a weather call option with strike K, payout
rate X and limit M, the expected payout, i.e. the expected loss of the
contract E(P)88, is given by:
(18)
where N is the cumulative normal distribution and μ and σ are the mean
and standard deviation defined by the historical mean and standard
deviation of the index data. The standard deviation of the contract (P)89
is given by:
(19)
For example, if the GDD index considered in Box 4 is normally
distributed with μ = 2567 and σ = 131, taking α = 25% the premium of
the call option given the closed form solution above and using
Equation 13 is:
Premium = E(P) + 0.25*(P) = $15,350 +0.25*$138,895 = $50,07490
Closed form solutions can also be derived for call and put options
using different underlying distributions, such as the kernel density91
and Gamma distribution92. Although the HDA method is more accurate
than HBA for computing expected and variable payouts93,94, and is
often simpler due to the availability of analytical formulas, it
assumes the underlying distribution is a correct representation of the
data. Fitting and putting too much emphasis on a distribution that
does not capture the higher moments of variability, for example, can
lead to underestimate of variability and therefore premium.
Monte Carlo Simulation
Index Simulation. Once a distribution is identified to represent an
index, constraints associated with the length of the historical data
record are no longer valid and thousands of realizations of the index
can be simulated, to estimate the contract statistics to any arbitrary
degree of statistical accuracy, by using the distribution to make
Monte-Carlo simulations. The Index Simulation (IS) method is a very
common method for pricing weather contracts. Index values can be
simulated statistically by drawing samples from the chosen
distribution to generate large numbers (years) of artificial index
values. The weather contract structure is applied to each of these
values to create a range of payout outcomes that can be used to
calculate the price of the contract. The IS method is particularly
good for cumulative contracts, such as GDDs, or contracts that depend
on several weather variables and where the correlation between these
variables can be included in the simulation process. An additional
advantage of the IS and HDA methods is that weather forecasts can be
incorporated in the pricing process though the E(P) and possibly (P)
terms by their dependence on E(I) and (I). The weather market
actively follows forecast information and will modify its estimates of
E(I) and (I) from historical information if necessary95.
Daily Simulation. Daily Simulation (DS) methods are one of the most
complex ways to price weather contracts. A statistical model is built
for daily meteorological variables and is used to create thousands of
years of artificial daily data. Index values can be calculated from
this data, the weather contract can be applied to each simulated index
value to create thousands of simulated payouts from which the expected
and variable payout statistics can be calculated. Building daily
simulation models that correctly capture the physical relationships
between many meteorological variables at many sites poses significant
scientific, mathematical and programming challenges96 and should only
need to be used for path-dependent contracts, e.g. knock-in or
knock-out options or exotic or non-linear structures that depend on
several variables or critical daily values. As these models involve
manipulating daily data, they tend to be much slower than the other
methods outlined above and, if built correctly, do not offer more
accuracy that the IS method for simpler structures such as cumulative
rainfall or GDD contracts97.
Market Pricing
Finally, for completeness, if underlying indices are the same or
well-correlated to indices that trade frequently in the secondary
over-the-counter and exchange-based derivative market, the prevailing
market price of traded contracts can influence the cost of the end
user contract through the terms E(P) or (P). For example, if
November-March GDDs correlate strongly with November-March HDDs
measured at the same station, then a market provider could use the
market-based HDD contract to hedge an end user GDD structure. If so,
the provider will want to account for the market price of the HDD
index in the pricing of the end user structure by adjusting the
theoretical structure cost by any extra costs or benefits incurred by
hedging98. This can be done by making the structure price independent
of model based estimates of μ and σ and allowing the market price of
the underlying HDD swap (μmarket) and the implied standard deviation
from the prices of HDD option contracts (σmarket) to be used instead
for the parameters of the index I when calculating E(P) or (P) via
HAD or IS methods. In other words, replacing the model estimates of μ
with γ*μmarket, and σ with γ*σmarket, where γ is the appropriate
conversion factor from cumulative HDDs to cumulative GDDs, as measured
at the station. Using the market as a benchmark also has an advantage
as the traded weather market incorporates weather forecast into its
prices. They therefore offer an indicator of how price estimates using
historical data could be modified to reflect forecast information99.
End User Perspective
On receiving a price quotation for a weather risk management solution
from a market provider, an agricultural grower or producer must decide
if, given the price, such a solution is the best strategy for the
business to manage its weather risk. Some of the advantages and
disadvantages of using a market-based risk management tool are
highlighted below for an end user to consider. There are many
technical and practical measures a grower can take to make his crops
more resilient to the vagaries of the weather, such as investigating
in better irrigation systems, new strains of seed or new farming
technologies. Likewise, an agricultural product sales company, for
example, may choose to diversify into other products in order to
reduce their overall exposure to a particular weather event. Although
such strategies will not be covered in this Chapter, the relative cost
and efficiency of choosing such approaches, over an insurance or
derivative weather based-solution, must also be considered by the end
user. Ideally the end user should focus on the most cost-efficient and
effective means for dealing with the weather risk it faces by
determining the optimal interaction of risk retention, risk transfer
and potential operational strategies, to create a comprehensive risk
management solution.
i. Revenue Volatility and Value-at-Risk
From an agricultural end user’s perspective, the cost of E(P) is
essentially already embedded in the business – it is the average
annual cost (loss) of weather inherent in running the business in
question, be it farming a crop in a particular region or selling a
specific agrochemical product. In other words, without protection the
grower or producer can expect to lose this amount on average each
year. Therefore the premium the grower or producer ultimately pays for
weather risk management product is only the risk margin charged by the
provider over the expected loss. This is illustrated by the schematic
below (Figure 7). By purchasing a tailored weather hedge an end user
receives a reduction of revenue volatility due to weather, but at a
cost – the risk margin. However, reducing the volatility at an
appropriate cost increases the return per unit risk, or the quality of
earnings of the end user (Box 5).
Obviously, the end user must also consider the efficacy of the weather
hedge and decide whether the risk management contract offers
protection in a worst-case scenario for his business. This can, to a
certain extent, be quantified with historical information. The
relevant question the end user should consider is whether the payout
from a risk management contract based on a weather index effectively
reduces the end user’s value-at-risk (VaR); in other words whether it
reduces the potential economic loss of the end user business expected
to be exceeded with a given probability within a given time horizon100.
A grower’s or producer’s VaR is an effective measure of the overall
vulnerability of the business to external shocks, be it price
movements or fluctuations in supply and demand for their product.
Weather protection that limits the potential downside revenue exposure
of a business reduces the end user’s overall VaR. Minimizing VaR also
has the associated cost – the risk margin – but it raises the question
as to whether a business could withstand extreme systematic shocks,
and their ramifications, without protection limiting losses in
catastrophic years.
The birth of the weather market has created an opportunity for a
business to protect itself from the impact of non-catastrophic weather
variations on its income statement. Previously, traditional insurance
products dealt primarily with losses that impacted the balance sheet,
through the protection of physical assets from damage due to
catastrophic weather. A business that protects its revenues and, as a
result, has a less volatile revenue stream may benefit by receiving,
for example, a lower cost of debt or increased access to credit and,
for public companies, potentially improved stock valuations or
stronger credit ratings101. Eliminating the uncertainty associated
with non-catastrophic weather-related risk allows an operation to
concentrate on its core business and focus on controllable targets and
growth. These benefits associated with reducing revenue volatility and
VaR, in relation to the effective cost of hedging, are considerations
for the end user. Just like the weather market providers, an end user
must also decide how it values risk in relation to return in the
context of its business. It must define how much risk it is willing to
hold and the budgeted cost at which it is willing to do so.
ii. Basis Risk
A major concern with index-based weather risk management products is
basis risk – the potential mismatch between contract payouts and the
actual loss experienced. On considering weather-index insurance as a
product for growers, Jerry Skees writes, “[t]he effectiveness of index
insurance as a risk management tool depends on how positively
correlated farm-yield losses are with the underlying area yield or
weather index.”102 As with the regulatory concerns regarding the
definition of insurance (Section 2), this statement relates to the
question of whether insurance based on a weather index can substitute
a traditional crop insurance policy and indemnify the grower for his
losses.
Basis risk is a concern for all weather variables but it is
particularly important for rainfall, which exhibits a high degree of
spatial and temporal variability. For example a weather station on
which a weather contract is based may not experience the same rainfall
patterns or totals during the calculation period as the locations an
end user wishes to protect. For this reason contracts based on hail
are not products that are offered by weather market providers; hail is
a highly localized meteorological phenomenon, although it can be
indexed to an observing weather station, it may not be an effective
risk management strategy for an end user. Although historically an
index and losses may correlate strongly – showing than an index could
be used as an underlying trigger to indemnify losses in an insurance
contract (Section 2) – a good correlation is not a guarantee that the
underlying contract payout will match the actual loss experienced.
Basis risk therefore – which can often be minimized by effective or
intuitive structuring and by using local stations103 – is always an
issue when dealing with an index-based risk management solution. A
potential basis risk outcome can be quantified by using historical
data, however the key point to consider, as outlined above, is the
efficacy of the hedge and the effective reduction the insured party’s
overall operational VaR104 (Box 5).
Box 4: The Corn Grower’s Weather Hedge
On receiving the grower’s price request, the broker contacts a company
that he knows actively sells weather risk management products. The
company already has historical weather data from Corntown Airport from
the NWS going back for 30 years, although it is not a station on which
they have written a weather contract on before. The data from the
Corntown Airport is good, with no gaps or obvious discontinuities
(Section 4). The weather structurer at the company computes the
cumulative MGDDs from May 6th – September 15th – as specified by the
broker – for each year in the historical data set. The structurer
observes that there is a significant warming trend in the data –
recent MGDD values have been higher than values in the past – and
therefore detrends the 30 MGDD index values to make the older data
consistent with today’s warmer temperatures (Box 6). The average value
of the MGDD index using 30-years of detrended data is E(I) = 2567 with
a standard deviation of σ(I) = 131.
The structurer then applies the weather insurance contract to each of
the 30 detrended MGDD index values to create a historical time-series
of contract payouts. He finds the average payout of the contract is
E(P) = $21,303 with a standard deviation σ(P) = $54,666. He takes α =
25% and therefore calculates a premium to be:
Premium = $21,303 + 0.25*$54,666= $34,970
He decides to quote the broker a price of $34,970 for the contract105.
Box 5: The Corn Grower’s Weather Hedge
The grower receives the quote from the broker for $34,970 for the
weather contract. He considers how this will impact the overall
revenue performance of his business and asks the postgraduate student
from the local Agricultural University, who helped him construct the
MGDD index, to assist him with the analysis. They study his past seven
years of yield data and the corresponding MGDDs values for each of
those years (Figure B). They then apply the grower’s corn supply
contract specifications and the weather hedge to each year to see what
would have happened.
Figure B: Historical Contract Payouts and Farm Yields
Table D: Corn Grower’s Expect Revenue With and Without Weather
Contract
Year
Yield (bu/hct)
MGDDs
Total Farm Production (bu)
Hedge Payout ($)
Net Revenue without Hedge ($)
Net Revenue with Hedge ($)
1998
263.4
2551
395,100
-34,970
882,850
847,880
1999
455.2
2651
682,800
-34,970
1,957,000
1,922,030
2000
145.5
2249
218,250
228,700
263,875
492,575
2001
510.5
2602
765,750
-34,970
2,164,375
2,129,405
2002
317.1
2399
475,650
17,200
1,164,775
1,181,975
2003
580.2
2649
870,300
-34,970
2,425,750
2,390,780
2004
481.6
2550
722,400
-34,970
2,056,000
2,021,030
They decide to ignore 1998, where farm production was low but the
hedge didn’t payout as they know there were other factors that
contributed to the low yield on the grower’s farm that year, that were
not temperature related. They compute the average revenue expected
from both strategies using data from 1999-2004: on average the grower
expects to make $1,671,963 when not weather hedging and $1,689,633
when hedging, despite paying an annual premium of $34,970. They then
study the deviations from the expected revenue level for both
strategies:
Table E: Deviation in Expected Revenue Under Two Risk Management
Strategies
Year
Deviation from Expected
Without Hedge ($)
Deviation from Expected
With Hedge ($)
1999
285,038
232,398
2000
-1,408,088
-1,197,058
2001
492,413
439,773
2002
-507,188
-507,658
2003
753,788
701,148
2004
384,038
331,398
Expected Revenue
1,671,963
1,689,633
Worst Case Revenue
263,875
492,575
Value-at-Risk
1,408,088
1,197,058
Expected Revenue/VaR
119%
141%
The grower is further encouraged when he sees the superior risk/return
characteristics of the weather hedging strategy over the unhedged
strategy. By entering into a weather contract for the past six years
the grower would have reduced his Value-at-Risk, which he defines to
be the difference between his expected revenue and his worst case
year, by over $200,000, resulting in a 32% increase in the risk-reward
ratio, i.e. his business would have generated a higher return for less
risk under the weather hedging strategy. Although he knows the MGDD
index does not correlate perfectly with his risk, he is satisfied with
the performance of the index as a base for hedging his corn production
in the past six years.
However, the postgraduate is not happy with this analysis and decides
to investigate the impact of weather on the grower’s revenues further.
He looks at all 30-years of historical cumulative MGDD values for May
6th – September 15th at Corntown Airport weather station and notices
that there is a warming trend in the data, making farming of corn more
difficult in the past due to cool summer temperature conditions. The
postgraduate decides to detrend the historical MGDD data by using a
linear least squares regression to adjust the older data and bring it
in line with current warmer levels. Although he does not have actual
production data for the grower’s farm for 30 years, he is satisfied
with the cumulative MGDD-yield relationship he established earlier and
uses the detrended MGDD data and Equation (b), to infer the yields the
grower would have expected to produce on his farm in the past 30 years
given the weather conditions in those years and his current farming
techniques. The postgraduate then applies the grower’s corn supply
contract specifications and the weather hedge to each detrended year
to see what would have happened and compares the statistics for both
strategies over the 30 scenarios. He calculates the average revenue,
standard deviation of revenue and Value-at-Risk for both strategies
and makes a table of the results to show the grower.
Table F: Revenue Statistics Under Two Weather Risk Management
Strategies
Statistics
Without Hedge ($)
With Hedge ($)
Expected Revenue
2,199,991
2,186,324
Worst Case
471,457
658,188
Value-at-Risk
1,728,534
1,528,136
Standard Deviation in Revenue
862,411
824,954
Expected Revenue/VaR
127%
143%
The grower sees that the expected revenue is less for the strategy
where he hedges his business risk but the postgraduate explains that
this is because he pays an annual premium of $34,970 to the insurance
company each year for the weather hedge. The grower understands that
the company must be charging more for the contract than the expected
or fair value. However, the grower notices that his worst-case revenue
year is almost $200,000 less under the weather hedging strategy and
that the standard deviation in his revenues under that strategy is
also reduced. He also notes the superior risk/return characteristics
of his business portfolio – a 16% increase in the risk-reward ratio –
under the weather hedging strategy as compared to the unhedged
strategy. Given these benefits the grower chooses to hedge his weather
exposure with the weather derivative contract.
Figure C: Distribution of Expected Revenues
4. Weather Data
===============
Data Requirements
-----------------
In order to implement a successful weather risk management program,
the data used to construct the underlying weather indices must adhere
to strict quality requirements, including:
1.
Reliable and trustworthy on-going daily collection and reporting
procedures.
2.
Daily quality control and cleaning.
3.
An independent source of data for verification (e.g. GTS weather
stations).
4.
A long, clean and internally consistent historical record to allow
for a proper actuarial analysis of the weather risks involved – at
least 30 years of daily data is ideally required.
The premium associated with weather risk management strategies is
based on a sound actuarial analysis of the underlying risk. An
appropriate premium given the probability and severity of specific
weather events will be charged by the commercial risk-taker, hence the
quality of historical and on-going weather data is paramount. Nearly
all weather contracts are written on data collected from official
National Meteorological Service weather stations; ideally, these are
automated stations that report daily to the GTS – the World
Meteorological Organization’s (WMO) Global Telecommunication System –
in internationally recognized standard format that then undergo
standard WMO-established quality control procedures.
In addition to defining financial or insurance terms, all weather
contracts must also include instructions on how to determine and
adjust weather data, for example in the event that weather data is not
recorded or unavailable from the specified source during the
calculation period. Financial contracts that trade in the secondary
OTC weather market are usually subject to fallback methodology
specifications106 which identify a nearby “fallback” station to be
used in the event of missing data from the primary reference weather
station (RWS) and detail exactly how fall-back station data will be
adjusted to infer and in-fill the missing data from the RWS. In cases
where fallback stations are not available other methodologies or
provisions must be outlined in the contract terms.
End user’s without access to weather data satisfying the above
criteria or where the spatial coverage of a National Meteorological
Service’s weather station network may not be sufficient to fully
represent an end user’s weather risk profile, may not able to benefit
from weather risk management solutions. However, there are potential
alternatives to data collected from ground-based observatories that
could be used to structure risk management products, which will be
outlined below.
Data Sources
============
i. Weather Stations
All contracts traded in the active secondary OTC derivative market are
based on climatic weather data collected and published by the National
Meteorological Service (NWS) of the country in question. Each weather
station in the global NWS network has a unique WMO ID number, which is
used for international identification, as well as a reference
latitude, longitude and elevation. Stations are generally manned by
NWS staff or volunteers who are trained by the NWS and whose equipment
is certified and maintained to the NWS to WMO standards. NWS weather
stations produce SYNOP reports, observations that are made at
internationally agreed times by all meteorological observers. The
regulations and practices are set by the WMO and adhered to by all
NMSs. SYNOP reports – covering elements such as temperature, wind
speed, rainfall, sunshine hours, humidity and atmospheric pressure –
are generally made at 3-hourly intervals to monitor real-time
conditions. NWSs then communicate this data from specific stations in
their observing network to the GTS, for dissemination to the global
weather observing and forecasting community. There are well over 8000
SYNOP stations reporting from sites around the world. SYNOP summaries,
for example for temperature and rainfall, are reported at the
following times:
*
Minimum Temperature is reported at 0600 GMT for the previous 12
hours.
*
Maximum Temperature is reported at 1800 GMT for the previous 12
hours.
*
Rainfall is reported at 0600 and 1800 GMT for the previous 12-hour
periods.
Climate data are daily summary reports of variables such as minimum,
maximum and average temperature, rainfall or sunshine hours from a
station. The reporting times vary from country to country and there is
no internationally agreed standard. For example in the UK values are
recorded for the period 0900-0900 GMT, in Germany 0730-0730 GMT, in
France 0600-0600 GMT, in Finland 1800-1800 GMT for temperatures and
0600-0600 GMT for rainfall107. Historical climate data is produced by
a NWS from the hourly SYNOP data after an internal quality control
process. For example108, each element is automatically checked: to see
that it falls within acceptable limits; against other reference data
to ensure consistency, such as checking that Tmin temperature is less
than Tmax temperature; for differences between nearby stations; for
values that fall outside the climatological extreme values for a
station; for large step changes between 3-hourly reports. Any values
that are flagged as being suspicious in the checks described above are
then checked by climate experts within the NWS, who will either
correct or reject a value based upon the evidence available. Similar
automatic checks are also performed on SYNOP data, as errors can
indicate faulty sensors or incorrectly submitted reports. It is only
after the above checks have been carried out that the climatic dataset
is released. For this reason the terms of a weather contracts, except
CME contracts, also include a 40-day correction period, that allows
for changes by the reporting agency in the data as a result of the
quality control process. The CME must settle positions on a daily
basis and use data provided by EarthSat109, who produce climatic data
values from NWS SYNOP data within a day, for CME contract settlement.
Historical climate and SYNOP data, and daily up-dates, can be
purchased from each NWS, a list of which can be found on the WMO
website. For example in the U.S. the primary source of weather data is
the National Climatic Data Center, in the UK weather data can be
purchased from Weatherxchange110, a joint venture with the UK Met
Office set up to support the European weather derivatives market.
Weatherxchange provides quality-controlled historical climate and
SYNOP data sets across UK and has distribution rights to data from
several NWS across Europe including Germany, Italy, France,
Netherlands, Austria and Spain. Data can also be purchased from
private data vendors, such as Risk Management Solutions/EarthSat111
and Applied Insurance Research (AIR)112. Private vendors often offer
additional value-added services such as data cleaning and adjusting
(see below).
ii. Reanalysis and Satellite Products
It is also worth mentioning that other weather datasets exist, that
could potentially be used for certain weather risk management products
and contracts if weather station data is not available or not
representative of the risk.
NCEP-NCAR Reanalysis. The NCEP-NCAR Reanalysis113 is a joint project
between the U.S. National Centre for Environmental Prediction (NCEP)
and the National Centre for Atmospheric Research (NCAR) to produce a
40-year record of global atmospheric analyses using a data
assimilation system that is kept unchanged over the reanalysis period
1958-1997. An identical Climate Data Assimilation System using the
same frozen analysis/forecast system has been used to continue to
perform data assimilation to date, to ensure the continuation of the
analysis. The reanalysis has a horizontal resolution of approximately
210km, with 28 vertical levels, and is a complete, consistent and
continuous gridded daily global dataset of all atmospheric variables
(surface and air temperature, precipitation, wind speed, pressure,
humidity) from 1958. The data is available for free download114 from
NCEP-NCAR, and although it has a low-resolution, it may be appropriate
for large-area weather exposures that are not covered by a weather
station network. The European Centre for Medium Range Forecasting
(ECMWF) has recently produced a higher resolution 40-year reanalysis115,
although it is not yet updated on an ongoing basis. The use of a
constant and consistent data assimilation system implies the dataset
would be an ideal base for pricing weather contracts, however the
low-resolution makes the reanalysis inappropriate for small-scale,
localized risk.
Satellite Data. Another alternative to weather-based indices is to use
satellite-based products to measure the pertinent weather parameters
traditionally measured using ground observatories. Two strong
candidates for agriculture include satellite-derived precipitation
estimates and Normalized Difference Vegetative Index (NDVI) satellite
readings. Current satellites offer high-resolution, albeit expensive,
data; NDVI data, for instance, could be used to monitor crop
“greenness” and therefore crop development and biomass. However,
satellite-based products are not often used in the weather market due
to their short and inconsistent historical data lengths; the first
generation of earth observing satellites were launched in 1979,
however calibrating older low-resolution data – through the
generations of improved versions – to data from the current satellites
is not straightforward. Nonetheless, both Spain116 and Alberta, Canada117
have recently launched drought insurance programs for forage based on
NVDI indices. Risk management products against tropical cyclones –
whose strength and location are measured and monitored using real-time
satellite data – are also available in the weather insurance industry.
The use of satellite data has also been recently discussed in the
broader context of traditional agricultural insurance products118.
Cleaning and Adjusted Data
==========================
Despite NWS quality control procedures data from some meteorological
observing stations may still have missing and erroneous values.
Stations may also have undergone instrumentation and/or station
location changes, which can introduce systematic changes to a
historical dataset. For instance, if a station was moved from a rural
to an urban location it may be several degrees warmer in the new
location and therefore there will be an artificial jump in the
station’s historical temperature record. Records of station or
instrumentation changes are usually kept by the NWS for each weather
station. Therefore in order for data to be used for pricing weather
risk management products, the raw data should be cleaned to correct
for errors and missing values, and checked and perhaps adjusted for
non-climatic inhomogeneities that could make the historical data
unrepresentative of current values. The methods of cleaning and
adjusting data often involve statistical procedures beyond the scope
of this Chapter. However an awareness of the possible need for
cleaning and adjusting of data is recommended and the approaches used
are briefly outlined below. Cleaned and adjusted datasets can also be
purchased from private vendors with proprietary data estimation
models, such as RMS and AIR.
Cleaned Data. Creating cleaned datasets involves identifying and
correcting erroneous values in historical weather datasets and
in-filling missing data with realistic values using appropriate
statistical techniques where necessary. The first steps are the same
as the NWS quality control procedures outlined above: all observations
are screened for physical inconsistencies and erroneous values by
comparing the data against itself and against alternative data sources
(e.g. SYNOP, hourly data, climate reports, station climatology and
surrounding stations). If missing data cannot be recovered (e.g. from
SYNOP data) or if data is found to be erroneous, the value is flagged
and filled with an estimated value. A common method used to construct
an estimate is to employ regression equations calculated from periods
of overlapping data with the n best correlated neighbour stations. A
weighted average of the estimates is used to calculate the missing
data value at the target station. The weights are set according to the
correlation coefficient between the target station and the surrounding
neighbours. This method can be used to both clean data and to extend
data records. Sometimes SYNOP or hourly data can also be used to
reconstruct missing or erroneous values.
Adjusted Data. Raw meteorological observations sometimes exhibit
non-climatic jumps in the historical record due to movements in the
measurement station, changes in the time of measurement or changes in
the equipment used to make the measurements. Often such
discontinuities do not significantly affect the historical record but
in some cases discontinuities can introduce artificial trends to the
data or impact the variance or the average value of readings.
Statistical procedures based on neighbouring stations exist to
identify significant discontinuities and account for these changes in
cleaned meteorological data records119,120. The challenge in these
methods is to correct for the artificial discontinuity without
altering the natural weather variability measured by the station.
Detrending Data
===============
Meteorological data often contains trends that arise either due to
natural climate variability, urban heating effects or the impact of
global warming. Irrespective of the cause, in some circumstances it
may be useful to be able to remove such trends from the data. Such a
procedure is known as detrending. The aim of detrending data for
pricing weather risk is to obtain better estimates or forecasts of
E(I), σ(I) and VaRX(I) from the historical data for pricing weather
contracts. Warming trends, for instance, can significantly impact the
defining parameters of the underlying data. By not accounting for such
trends E(I) may be significantly under-estimated and σ(I)
over-estimated, which can lead to mispricing of contracts which settle
on future data. Many different mathematical methods exist for
detrending data, each based on a different set of assumptions.
As well as choosing the method there are two further points to
consider when detrending data. Firstly, the underlying data must be
prepared in a selected format: daily, monthly or annual averages of a
meteorological parameter can be detrended and then the new detrended
historical values of an index can be computed; or the historical
values of the index itself can be detrended. Secondly, the number of
years of historical data or index values to be considered in the
detrending process must be selected121. Detrending data using the same
method but a different number of years, for example 30-years versus
40-years, can lead to significantly different results.
In essence the aim of detrending is to statistically model the
underlying process by decomposing a dataset into a deterministic trend
and a stochastic noise term around the trend:
D(t) = Y(t) + ε(t), ε(t) ~N(0,σ2) (20)
where D(t) is the process represented by the dataset, Y(t) is the
deterministic and therefore predictable component, ε(t) is a normally
distributed noise component with a mean of zero and standard deviation
σ and t is unit time. Determining how much of the historical data
variability is attributed to Y(t) gives an indication of how well a
particular model represents the underlying data. The method and
approach chosen for detrending data can be highly subjective and the
decision to detrend or not to detrend should be informed by some
underlying criteria122. For example, choosing a detrending method that
is better at predicting future data values than another method, or
than not doing anything at all, is preferable to a method that
increases the uncertainty in predicting future values. The performance
of different methods can be compared by considering characteristics of
the distribution of errors in the predictions they make. By using the
historical data to back-test various detrending methods and
approaches, estimates of the uncertainty around the trend can be found
which can inform the error associated with a particular method for
estimating future values.
However, identifying trends and their cause is itself a subjective
process and care should always be taken to check the sensitivity of
detrending results to the underlying method used. Cross-checking
several detrending methods and approaches and visually sense-checking
the data is always recommended. The weather market often uses the
10-year average of an index as a quick first-guess estimate for E(I).
The simplest and most commonly used method for detrending data,
polynomial detrending, is outlined below as an example.
Polynomial Detrending. The aim of this method is to fit a polynomial
function of time to a meteorological dataset. The polynomial function
is defined as:
Y(t) = m0 + m1 t + m2 t2 + m3 t3 + … + mp t p (21)
where t is time. For example, if the underlying dataset is composed of
40 historical index values from 40-years of weather data, t will be in
years. The constants mi are chosen to minimize the root mean squared
error R2 of the vertical deviation of n meteorological data points
from the trend line, where R2 is given by123:
(22)
The simplest polynomial trend is when p = 1, a linear trend which fits
a straight line through a set of data points:
Y(t) = m0 + m1 t (23)
The intercept and slope m0 and m1 are estimated by the intercept and
slope of the least-squares regression line.
The standard error (SE) in the estimated slope parameter m1 and
intercept parameter m0 from the least-squares regression line are
given by124:
(24)
(25)
where i = 1, … n , n is the number of years of historical data
included in the regression and s is an estimate of σ, the standard
deviation of the noise term in Equation 20, given by:
(26)
The standard error of the linear model predicting an individual value
D(tX) at a time tX, for example in year n+1, is given by125:
(27)
The t-statistic126 of the linear slope term m1 can be used to
determine whether or not the linear trend is statistically significant
and is defined as:
(28)
The t-statistic for that coefficient is the ratio of the coefficient
to its standard error. The t-statistic can be tested against a
Student’s t-distribution with n-2 degrees of freedom to determine how
probable it is that the true value of the coefficient is zero and thus
how significant the fit is. The r2 value is the fraction of the total
squared error that is explained by the linear model and is an
indicator of the predicative power of the model. The r2 value is
calculated as follows:
(29)
and is the square of the correlation coefficient, r, between the
linear model predictions and actual data observations. A simple method
to test the null hypothesis that the correlation coefficient is zero
can be obtained by using a Student's t-test with n-2 degrees of
freedom on the t-statistic:
(30)
where n is the number of years of historical data considered. By
comparing values for SEy, r2 and the statistical significance of m1
for a given confidence level, decisions can be made as to whether a
linear trend actually describes the data well and the optimal number
of years of data n to be considered in the calculation. The detrended
historical dataset, Ð(t), will then used to calculate new values of
the index I and therefore to calculate revised estimates of E(I), σ(I)
and VaRX(I) for pricing. Values of Ð(t) are given by:
Ð(ti) = D(ti) – Y(ti) + Y(tn), i = 1 … n (31)
for all n years that are included in the detrending calculation, where
n is the most recent year (Box 6).
Often increasing the parameter p creates a better data fit, as higher
order polynomials capture higher frequency variations in the data.
However over-fitting data is a potential danger of all trend-fitting
techniques. By arbitrarily increasing p, high-frequency fluctuations,
essentially the noise in the underlying historical data record, can be
reproduced by the model, which will have little predictive power for
future data. The underlying physical nature of a higher-order
polynomial should also be questioned and therefore it is often best to
fit a simple linear trend to data instead of assuming higher-order
processes. Examples of other detrending techniques include the moving
average127, LOESS128 and low-pass filter129 methods.
Box 6: The Corn Grower’s Weather Hedge
On analyzing the historical MGDD record from Corntown Airport weather
station the structurer at the company responsible for pricing the
request from the broker realizes that there is a strong warming trend
in the data. Temperatures were cooler and hence cumulative MGDD values
were lower in the 1970s compared to the late 1990s and early 2000s.
Therefore in order to get a better estimate of the weather insurance
contract payout statistics, the structurer decides to detrend the raw
MGDD record, D(ti). He chooses a first-order polynomial function,
Y(t), to model the trend in the MGDDs:
Y(t) = m0 + m1 t (e)
He fits a least-squares regression line to the data that minimize the
root mean squared error of the data points around the trend line. The
intercept and slope, m0 and m1, are estimated by the intercept and
slope of the least-squares regression line and are found to be m0 =
-12346 m1 = 7.4413. The r2 of the fit is 20.1%, which implies that the
linear trend explains 20% of the overall interannual variability of
the MGDD index. He computes standard error in the m1 estimate and
therefore the t-statistics for the coefficient m1:
tstatisticm1 = 7.4413 / 2.808 = 2.65
The t-statistic is tested against a Student’s t-distribution with n-1
degree of freedom to determine how probable it is that the true value
of the coefficient is greater than zero. The t-statistic is
significant at the 99.3% confidence level, i.e. the probability that
the true value of the coefficient is zero is 0.7%. Therefore the
structurer is happy to use the linear model to detrend the historical
MGDD data.
The detrended dataset Ð(ti) is constructed by adjusting each
historical value by the amount predicted by the linear trend model,
i.e.:
Ð(ti) = D(ti) – Y(ti) + Y(t2004), i = 1975 … 2004 (f)
for all 30 years that are included in calculation. The average value
of the MGDD index, using 30-years of raw historical data, is E(I) =
2459 with a standard deviation of σ(I) = 146. The average value of the
MGDD index, using 30-years of detrended data, is E(I) = 2567 with a
standard deviation of σ(I) = 131. The structurer then applies the
weather insurance contract to each of the 30 detrended MGDD index
values to create a historical time-series of contract payouts. He
finds the average payout of the contract is E(P) = $21,303 with a
standard deviation σ(P) = $54,666. He takes α = 25% and therefore
calculates a premium to be:
Premium = $21,303 + 0.25*$54,666= $34,970
He compares this to what the premium would have been if he had not
adjusted for the warming trend in the data. He finds the average
payout of the contract, based on raw MGDD values, is E(P) = $69,068
with a standard deviation σ(P) = $114,809 which would imply a premium
of:
Premium = $69,068 + 0.25*$114,809= $97,770
T
he warming trend at Corntown Airport is decreasing the risk of cool
summers in the area and hence is reducing the premium of a weather
hedge designed to protect against this risk for the grower.
Figure D: MGDDs at Corntown Airport Weather Station
5. Further Reading
The emerging weather risk market clearly offers new risk management
tools and opportunities for agriculture. The aim of this chapter was
to briefly illustrate how an end user in the agricultural industry
could use a market-based solution to mitigate the financial impact of
weather on its business operations. The key steps for designing a
weather risk management program outlined above involve: identifying
and quantifying the weather risk; structuring a weather risk
management solution that best protects the end user; executing a the
contract in optimal form given the local regulatory framework. These
processes necessitate obtaining, analyzing and possibly cleaning,
adjusting and/or detrending historical weather data, to understand the
nature of the underlying weather risk and its financial impact on a
business, in order to structure an appropriate risk-transfer or
risk-smoothing solution.
Information for this chapter was taken from a wealth of literature
that has been written on the subject of weather risk management and
interested readers are strongly recommended to refer to these texts
for further information and discussion. An excellent in-depth
introduction to the weather market can be found in “Weather Risk
Management: Markets, products and applications” (Banks, E. ed, 2002)130.
More general reading of weather risk and weather risk management can
be found in “Climate risk and the weather market” (Dischel, R. 2002)131
and “Insurance and weather derivatives” (German, H. 1999)132. The
Social Science Research Network at http://www.ssrn.org contains a
large quantity of papers and articles on aspects of weather
derivatives from analytical pricing methods, simulation models,
detrending methods and the use of forecasts. The papers written by Dr.
Stephen Jewson are particularly recommended and can be found on
http://www.stephenjewson.com. Another good sources of articles and
information on weather derivatives and the market is the Artemis
website at http://www.artemis.bm and the industry body the Weather
Risk Management Association at http://www.wrma.org. The Guaranteed
Weather website has a wealth of case studies and weather risk
management examples at http://www.guaranteedweather.com/casestudies.php.
Information on weather risk management in the developing world can be
found at http://www.itf-commrisk.org.
Figure 1: Notional Value of All Weather Contracts in $US
F
igure 2: Percentage of Total Weather Contracts by Location (Excluding
CME Trades)
Figure 3: Potential End user Market by Economic Sector 2003/2004
Figure 4: Percentage of Total Weather Contracts by Index (Excluding
CME Trades)
Figure 5: Call Option Payout Structure and Wheat Grower’s Losses
F
igure 6: Collar Payout Structure and Agrochemical Company’s Deviation
from Budgeted Revenue
Figure 7: Schematic of a Business’s Historical Revenues and the Impact
of Weather Hedging
1 Joanna Syroka is a consultant for the World Bank’s Commodity Risk
Management Group working on developing weather risk management
projects in the developing world. Prior to joining the World Bank, she
worked as an analyst for Centrica Plc, one of the UK's largest utility
companies, responsible for developing quantitative weather and gas
risk management strategies for Centrica's trading and hedging
operations. Joanna holds a PhD in Atmospheric Physics from Imperial
College, London.
2 Weather Risk Management Association, 2004, “How climate changes
affect the European Economy”
3 World Bank, 2004, “Anticipating Shocks in Low-Income Countries and
Managing Debt Risk Through Financial Instruments”
4 Auffret, P., 2003, “High consumption volatility: the impact of
natural disasters”, World Bank Working Paper 2962
5 Thomas Loster, Munich Re, “Risking Cost of Natural Disasters and
their Impacts on Insurance”, ProVention Consortium International
Conference, October 2004, Zurich, Switzerland
6 Commerce Secretary William Daley, 1998.
7 “Wet Spring, Cold Winter Halt Work”, 02/03/2004, Home News Tribune,
Central New Jersey
8 An excellent introduction is given in Clemmons, L., Chapter 1,
Banks, E., Ed., 2002, “Weather Risk Management: Markets, Products and
Applications”, Palgrave, New York.
9 Duke Energy, Third Quarter 2003 Results
10 Energy East Corporation, 2003 Financial Results
11 Southwest Gas Corp., Annual Report to Shareholders, 2003
12 Coca-Cola Enterprises Inc., Third-Quarter 2004 Results
13 Jake Ulrich, Managing Director Centrica Energy Risk Management
Group, Press Release November 2002
14 Press Release, May 26 2004.
15 The Times, 29 December 2003, London.
16 Leisure and Brewing Analyst, UBS Warburg, 1999
17 PWC Survey 2003 and 2004.
18 The publication Energy Risk survey respondents estimate that the
market is worth around 45% more in 2004. WRMA’s survey relies on
figures from 19 companies – all members of the Washington DC-based
organization. Some large weather trading operations, such as Deutsche
Bank and Calyon, are not WRMA members and therefore the true size of
the market is hard to determine.
19 Most energy-related weather transactions are based on temperature
indices such as Heating Degree Days (HDDs) and Cooling Degree Days
(CDDs), designed to correspond to fluctuations in demand for gas
(heating) and power (cooling, i.e. air conditioning).
20 http://www.sfb.nl/index.html
21 In 1999 the Chicago Mercantile Exchange (CME) began listing and
trading standard weather futures and options contracts on temperature
indexes. They now list 22 locations in the U.S., Europe and Japan.
22 Hess, U., 2003, “Innovative Financial Services for Rural India”,
World Bank Agriculture and Rural Development Working Paper 9
23 “Hedging the Horsemen”, The Economist, 11 December 2004
24 Hess, U. and J. Syroka, 2005, “Weather-based insurance against
covariate shocks in Southern Africa: Focus Malawi”, World Bank working
paper, forthcoming 2005.
25 PWC Survey 2004
26 “Freeze Risk to Citrus Crops”, www.guartanteedweather.com
27Stoppa, A. and U. Hess, 2003, “Design and Use of Weather Derivatives
in Agricultural Policies: The Case of Rainfall Index Insurance in
Morocco”, International Conference on Agricultural Policy Reform and
the WTO: Where are we Heading, Capri, Italy, 23-26 June, 2003
28 “The Feasibility Of A Derivative For The Potato Processing Industry
In The Netherlands”, www.guartanteedweather.com
29 “The Effects Of Temperature Stress On Dairy Production”,
www.guartanteedweather.com
30 California Association of Winegrape Growers, http://www.cawg.org/
31 Example taken from “Brewery Barley Risk Management”,
www.guaranteedweather.com
32 From “Application of Weather Derivatives in the Agricultural
Chemicals Industry”, WRMA (www.wrma.org): “Development of many
organisms which cannot internally regulate their own temperature, is
dependent on temperatures to which they are exposed in the
environment. Plants and invertebrates, including insects and
nematodes, require a certain amount of heat to develop from one point
in their life-cycle to another, e.g., from eggs to adults. Because of
yearly variations in weather, calendar dates are not a good basis for
making management decisions. Measuring the amount of heat accumulated
over time provides a physiological time scale that is biologically
more accurate than calendar days.”
33 National Cotton Council of America, http://www.cotton.org/
34 “Application of Weather Derivatives in the Agricultural Chemicals
Industry”, WRMA (www.wrma.org)
35 Skees, J., P. Hazell and M. Miranda, 1999, “New Approaches to
Public/Private Crop Yield Insurance”,
World Bank Mimeo, Washington DC
36 Potential mismatch between insured party’s actual loss and the
weather contract payment.
37 http://www.fao.org/ag/agl/aglw/cropwater/maize.stm
38 Section 4 gives more information on the weather station and data
requirements and providers.
39 PWC Survey 2004
40 A HDD is calculated according to how many degrees an average daily
temperature varies below a baseline of 65 degrees Fahrenheit (18 deg
Celsius) and is defined as HDD = max( 0, 65 – T) where T is the daily
average temperature.
41 A CDD is calculated according to how many degrees an average daily
temperature varies above a baseline of 65 degrees Fahrenheit (18 deg
Celsius) and is defined as CDD = max( 0, T - 65) where T is the daily
average temperature.
42 Midwestern Regional Climate Center, IL, U.S.
http://mcc.sws.uiuc.edu/
43 From “Application of Weather Derivatives in the Agricultural
Chemicals Industry”, WRMA www.wrma.org
44 Neild, R. E. and J. E. Newman, “Growing Season Characteristics and
Requirements of the Corn Belt”, Purdue University,
http://www.ces.purdue.edu/extmedia/NCH/NCH-40.html
45 Neild, R. E. and J. E. Newman, “Growing Season Characteristics and
Requirements of the Corn Belt”, Purdue University,
http://www.ces.purdue.edu/extmedia/NCH/NCH-40.html
46 Byrne, D. H. and T. Bacon, “Chilling Accumulation: its Importance
and Estimation”, Dept. Of Horticultural Sciences, Texas A&M
University, College Station, TX 77843-2133
47 Byrne, D. H. and T. Bacon, “Chilling Accumulation: its Importance
and Estimation”, Dept. Of Horticultural Sciences, Texas A&M
University, College Station, TX 77843-2133
48 “Freeze Risk to Citrus Crops”, GuaranteedWeather Case Study,
www.guaranteedweather.com
49 Ministry of Agriculture and Food, Ontario, Canada
http://www.gov.on.ca/OMAFRA/english/crops/facts/85-116.htm
50 Private Communication: Adamenko, T.,“Agroclimatic Conditions and
Assessment of Weather Risks for Growing Winter Wheat in Kherson
Oblast”, July 2004, Ukrainian Hydrometeorological Centre, Kiev.
51 Private Communication: Adamenko, T.,“Agroclimatic Conditions and
Assessment of Weather Risks for Growing Winter Wheat in Kherson
Oblast”, July 2004, Ukrainian Hydrometeorological Centre, Kiev.
52Eftha, A., “Irrigation Management of Sugar Beets”, Agriculture, Food
and Rural Development, Government of Alberta
53 “Agriculture Industry Study”, Weather Risk Management Association,
www.wrma.org
54 Neild, R. E. and J. E. Newman, “Growing Season Characteristics and
Requirements of the Corn Belt”, Purdue University,
http://www.ces.purdue.edu/extmedia/NCH/NCH-40.html
55 Stoppa, A. and U. Hess, 2003, “Design and Use of Weather
Derivatives in Agricultural Policies: The Case of Rainfall Index
Insurance in Morocco”, International Conference on Agricultural Policy
Reform and the WTO: Where are we Heading, Capri, Italy, 23-26 June,
2003
56 Private Communication: Adamenko, T.,“Agroclimatic Conditions and
Assessment of Weather Risks for Growing Winter Wheat in Kherson
Oblast”, July 2004, Ukrainian Hydrometeorological Centre, Kiev.
57 Palmer, W. C., 1965, “Meteorological Drought”, Office of
Climatology of the U.S. Weather Bureau.
58 “Agriculture Industry Study”, Weather Risk Management Association,
www.wrma.org
59 Private Communication: Adamenko, T.,“Agroclimatic Conditions and
Assessment of Weather Risks for Growing Winter Wheat in Kherson
Oblast”, July 2004, Ukrainian Hydrometeorological Centre, Kiev.
60 Corbally, M. and P. Dang, Chapter 7, Banks, E., Ed., 2002, “Weather
Risk Management: Markets, Products and Applications”, Palgrave, New
York.
61 To be precise this definition describes a European Option, an
option that can only be exercised at the end of its life, at maturity.
In general, this is the most appropriate type of options on an
underlying weather index. Other types of options include: American
Options, an option that can be exercised at any time during its life;
Bermudan Options, an option that can be exercised on specific dates
during its life; and Asian Options, an option with a payout function
that depends on the average value of the underlying index during a
specified period.
62 Raspe, A., Chapter 12, Banks, E., Ed., 2002, “Weather Risk
Management: Markets, Products and Applications”, Palgrave, New York.
63 Source: CME
64 See footnote 39.
65 See footnote 40.
66 Thanks to Claude Brown, Clifford Chance, London, for his comments
and advice for this sub-section,
67 Raspe, A., Chapter 12, Banks, E., Ed., 2002, “Weather Risk
Management: Markets, Products and Applications”, Palgrave, New York.
68 International Swaps and Derivatives Association (ISDA),
www.isda.org. WRMA has worked closely with ISDA to produce long form
confirmations for standardized weather derivative contracts.
69 Raspe, A., Chapter 12, Banks, E., Ed., 2002, “Weather Risk
Management: Markets, Products and Applications”, Palgrave, New York.
70 “ISD2 Mandates Weather Derivative Regulation Review”, Clifford
Chance, May 2004.
71 “ISD2 Mandates Weather Derivative Regulation Review”, Clifford
Chance, May 2004.
72 Raspe, A., Chapter 12, Banks, E., Ed., 2002, “Weather Risk
Management: Markets, Products and Applications”, Palgrave, New York.
73 The federal agency created by U.S. Congress in 1975 to regulate
futures and options trading through its administration of the
Commodities Exchange Act, a federal act which regulates the futures
and options industries, requiring all futures and options to be traded
on organized exchanges.
74 These six point are taken from the Weather Risk Management
Association response to the National Association of Insurance
Commissioners (NAIC) Draft White Paper entitled, “Weather Financial
Instruments (Temperature): Insurance or Capital Markets Products?” The
paper and response can be found at www.wrma.org.
75 Weather Risk Management Association response to the National
Association of Insurance Commissioners (NAIC) Draft White Paper
entitled, “Weather Financial Instruments (Temperature): Insurance or
Capital Markets Products?” The paper and response can be found at
www.wrma.org.
76 OGC Opinion No. 2000-26 of the General Counsel, New York Insurance
Department, also noted in WRMA response to the National Association of
Insurance Commissioners (NAIC) Draft White Paper entitled, “Weather
Financial Instruments (Temperature): Insurance or Capital Markets
Products?” The paper and response can be found at www.wrma.org.
77 An excellent introduction to pricing weather risk can be found in
Henderson, R., Chapter 10, Banks, E., Ed., 2002, “Weather Risk
Management: Markets, Products and Applications”, Palgrave, New York.
78 Henderson, R., Chapter 10, Banks, E., Ed., 2002, “Weather Risk
Management: Markets, Products and Applications”, Palgrave, New York.
79 Information taken from the Partner Re website, www.partnerre.com
80 Henderson, R., Chapter 10, Banks, E., Ed., 2002, “Weather Risk
Management: Markets, Products and Applications”, Palgrave, New York.
81 Hull J. C., 2000, “Options, Futures and Other Derivatives”, 4th
ed., Prentice-Hall International
82 Henderson, R., Chapter 10, Banks, E., Ed., 2002, “Weather Risk
Management: Markets, Products and Applications”, Palgrave, New York.
83 Equation taken from Henderson, R., Chapter 10, Banks, E., Ed.,
2002, “Weather Risk Management: Markets, Products and Applications”,
Palgrave, New York.
84 Equation taken from Henderson, R., Chapter 10, Banks, E., Ed.,
2002, “Weather Risk Management: Markets, Products and Applications”,
Palgrave, New York.
85 More information about distribution fitting, can be found in:
Groebner, D. F. and P. W. Shannon, 1993, “Business Statistics: A
Decision-Making Approach”, 4th ed., Macmillan Publishing Company,
New York; Law, A. M. and D. Kelton, 1991, “Simulation Modeling and
Analysis”, 2nd ed., McGraw-Hill, New York; Walpole, R. E. and R. H.
Myers, 1993, “Probability and Statistics for Engineers and
Scientists”, 5th ed., Macmillan Publishing Company, New York.
86 McIntyre, R., 1999, “Black Scholes will do”, Energy, Power and Risk
Management, November 1999; Jewson, S., 2003, “Closed-form expressions
for the pricing of weather derivatives Part 1 - the expected payoff”,
http://ssrn.com/abstract=436262
87 Equation taken from Jewson, S., 2003, “Closed-form expressions for
the pricing of weather derivatives Part 3 - the variance payoff”,
http://ssrn.com/abstract=481902
88 As above.
89 Jewson, S., 2003, “Closed-form expressions for the pricing of
weather derivatives Part 3 - the variance payoff”,
http://ssrn.com/abstract=481902
90 The premium is significantly higher than the premium calculated in
Box 5 using the simple HBA method. The closed-form solution implicitly
takes into account the distribution of the underlying index and the
possibility of the contract payout reaching the $400,000 limit. The
HBA method can only take into account what has happen historically –
the maximum payout of the contract using the detrended MGDD data is
only $221,700 – therefore the latter method will under-estimate the
variability of the index, assuming the normal distribution adequately
represents the detrended MGDD data. This is one of the limitations of
the HBA method.
91 Jewson, S., 2004, “Closed-form expressions for the pricing of
weather derivatives Part 4: the kernel density”,
http://ssrn.com/abstract=486422
92 Jewson, S., 2004, “Closed-form expressions for the pricing of
weather derivatives: the expected payoff for gamma distributed
indices”, http://ssrn.com/abstract=576661
93 Henderson, R., Chapter 10, Banks, E., Ed., 2002, “Weather Risk
Management: Markets, Products and Applications”, Palgrave, New York.
94 Jewson, S., 2004, “Comparing the potential accuracy of burn and
index modelling for weather option valuation”,
http://ssrn.com/abstract=486342
95 Jewson, S., 2004, “Making use of the information in ensemble
weather forecasts: comparing the end to end and full statistical
modelling approaches”, arXiv:physics/0409097
96 Brody, D. C., J. Syroka and M. Zervos, 2002, “Dynamical Pricing of
Weather Derivatives”, Quantitative Finance, Vol. 2, No. 3, June 2002
97 Jewson, S., 2004, “Weather derivative pricing and the potential
accuracy of daily temperature modelling”,
http://ssrn.com/abstract=535122
98 Henderson, R., Chapter 10, Banks, E., Ed., 2002, “Weather Risk
Management: Markets, Products and Applications”, Palgrave, New York.
99 Dischel, R., 2000, “Seasonal weather forecasts and derivative
valuation” Energy Power and Risk Management Weather Risk Special
Report, August 2000.
100 Hull J. C., 2000, “Options, Futures and Other Derivatives”, 4th
ed, Prentice-Hall International
101 Malinow, M., Chapter 5, Banks, E., Ed., 2002, “Weather Risk
Management: Markets, Products and Applications”, Palgrave, New York.
102 Skees, J., 2003, “Risk Management Challenges in Rural Financial
Markets: Blending Risk Management Innovations with Rural Insurance”,
prepared for presentation at Paving the Way Forward for Rural Finance:
An International Conference on Best Practices June 2 – 4, 2003,
Washington DC.
103 Hess, U. and J. Syroka, 2005, “Weather-based insurance against
covariate shocks in Southern Africa: Focus Malawi”, World Bank working
paper, forthcoming 2005.
104 Hess, U., 2003, “Innovative Financial Services for Rural India:
Monsoon-Indexed Lending and Insurance for Smallholders”, World Bank
Agriculture & Rural Development Working Paper 9
105 See Equations 18 and 19, under “Historical Distribution Analysis”,
Section 3, for an alternative approach to pricing the corn grower’s
contract.
106 The Weather Risk Management Association (WRMA) provides a standard
of operation and business practices in the form of Standardized
Contracts/Confirms for weather derivatives and has developed Exposure
Calculation and Fallback language to include in financial contracts
(www.wrma.org).
107 Information taken from Weatherxchange Ltd., a joint venture with
the UK Meteorological Office, www.weatherxchange.com
108 Information taken from Weatherxchange Ltd., a joint venture with
the UK Meteorological Office, www.weatherxchange.com
109 www.earthsat.com
110 www.weatherxchange.com
111 www.rms.com, www.earthsat.com
112 www.air.com
113 Kalnay et al., 1996, “The NCEP_NCAR 40 year Reanalysis Project”,
Bull. Amer. Meteor .Soc, 77, 437-471.
114 http://www.cpc.ncep.noaa.gov/products/wesley/reanalysis.html
115 http://www.ecmwf.int/research/era/
116
117 “Ranchers Enter the Space Age”, Environmental Finance, March 2004.
118 “Earth Observation responses to Geo-Information Market Drivers”
Aon
http://www.aon.com/uk/en/risk_management/risk_consulting/eoreport1.jsp
119 Jewson, S., J. Hamlin and D. Whitehead, 2003, “Moving Stations and
Making Money”, Environmental Finance, November 2003; Boissonnade, A.,
L., Heitkemper and D. Whitehead, 2002, “Weather data: cleaning and
enhancement” In “Climate Risk and the Weather Market”, Chapter 5, Risk
Books, 2002.
120 Henderson, R., Y. Li and N. Sinha, Chapter 11, Banks, E., Ed.,
2002, “Weather Risk Management: Markets, Products and Applications”,
Palgrave, New York.
121 Jewson, S., 2004, “The relative importance of trends,
distributions and the number of years of data in the pricing of
weather options”, http://ssrn.com/abstract=516503
122 Jewson, S. and J. Penzer, 2004, “Weather derivative pricing and a
preliminary investigation into a decision rule for detrending”,
http://ssrn.com/abstract=618590
123 Weisstein, E. W., “Least Squares Fitting”, from MathWorld – A
Wolfram Web Resource,
http://mathworld.wolfram.com/LeastSquaresFitting.html
124 von Storch, H. and F. W. Zwiers, 1999, “Statistical Analysis in
Climate Research”, Cambridge University Press, Cambridge, UK
125 von Storch, H. and F. W. Zwiers, 1999, “Statistical Analysis in
Climate Research”, Cambridge University Press, Cambridge, UK
126 von Storch, H. and F. W. Zwiers, 1999, “Statistical Analysis in
Climate Research”, Cambridge University Press, Cambridge, UK
127 Henderson, R., Y. Li and N. Sinha, Chapter 11, Banks, E., Ed.,
2002, “Weather Risk Management: Markets, Products and Applications”,
Palgrave, New York.
128 Cleveland, W. S., 1979, “Robust Locally Weighted Regression and
Smoothing Scatterplots”, Journal of the American Statistical
Association, Vol. 74, pp. 829-836; Jewson, S., and J. Penzer, 2004,
“Following the Trend”, http://stephenjewson.com/articles/61.pdf
129 von Storch, H. and F. W. Zwiers, 1999, “Statistical Analysis in
Climate Research”, Cambridge University Press, Cambridge, UK
130 Banks, E., ed., “Weather Risk Management: Markets, Products and
Applications”, Palgrave, New York, 2002.
131 Dischel, R., ed., “Climate Risk and the Weather Market”, Risk
Books, 2002.
132 Geman, H., ed., “Insurance and Weather Derivatives”, Risk Books,
1999.
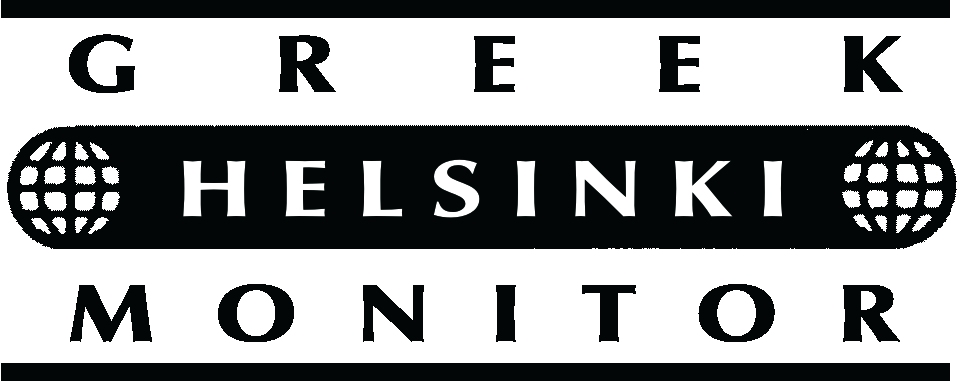
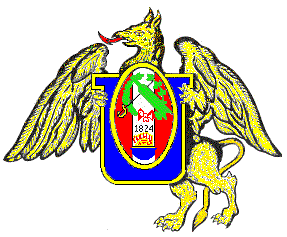
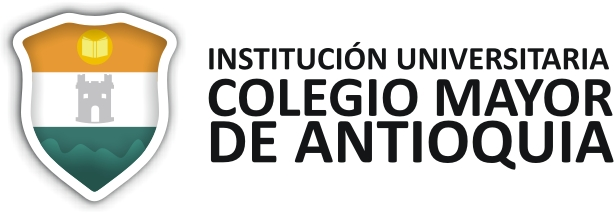
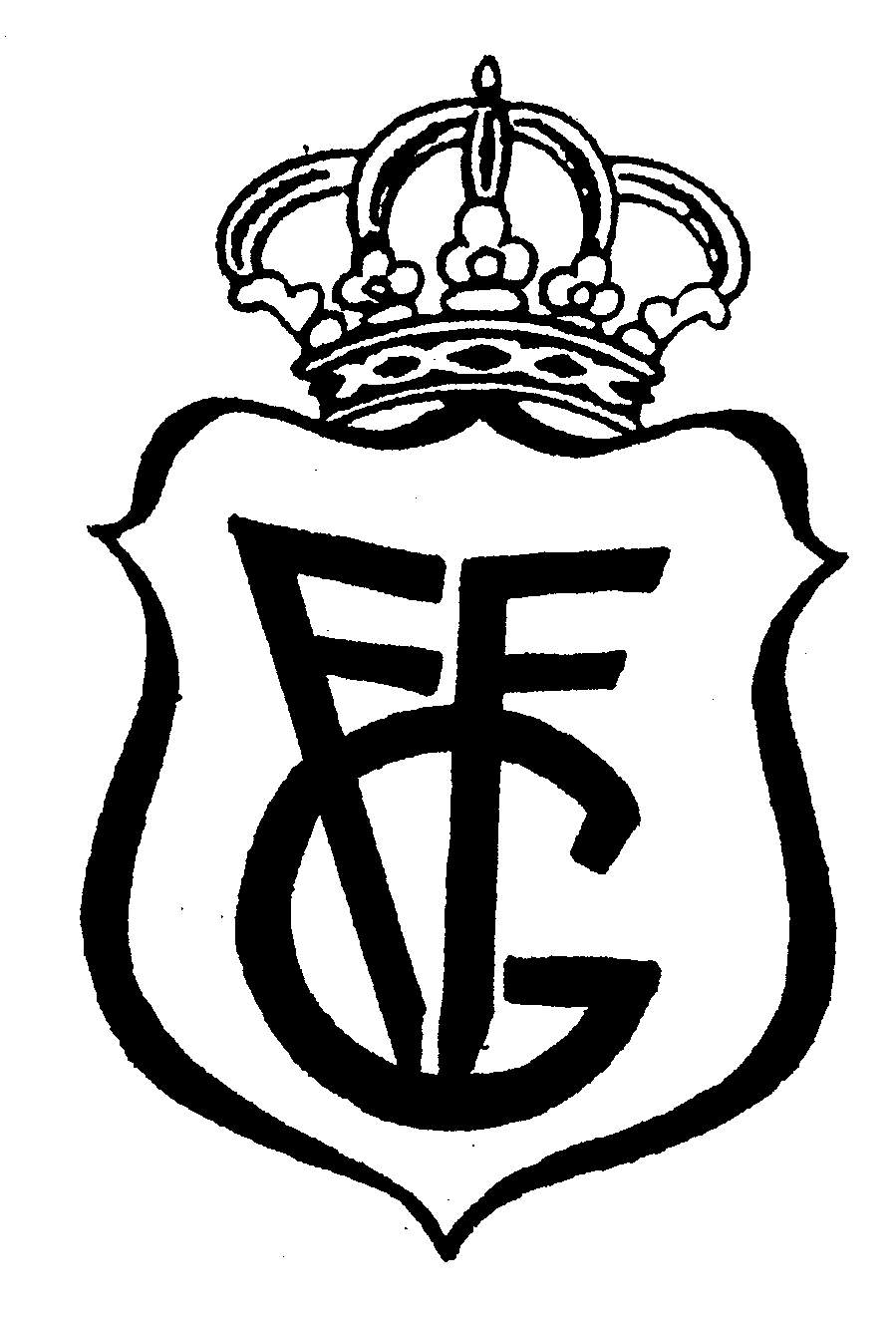
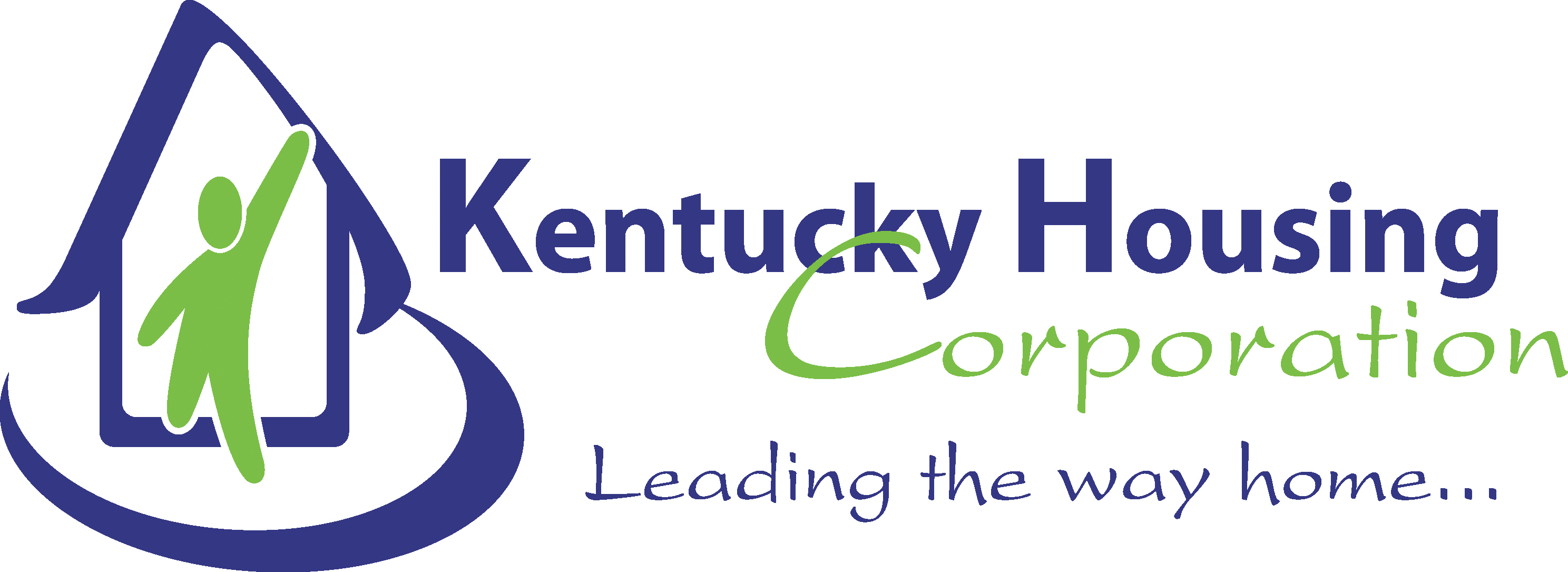
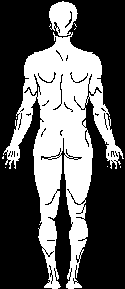
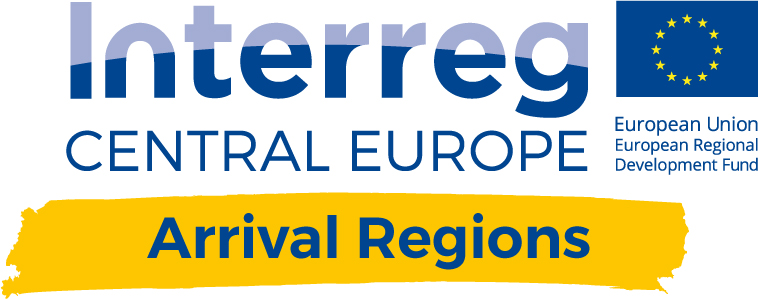
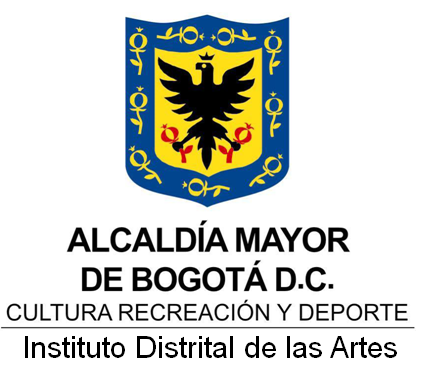

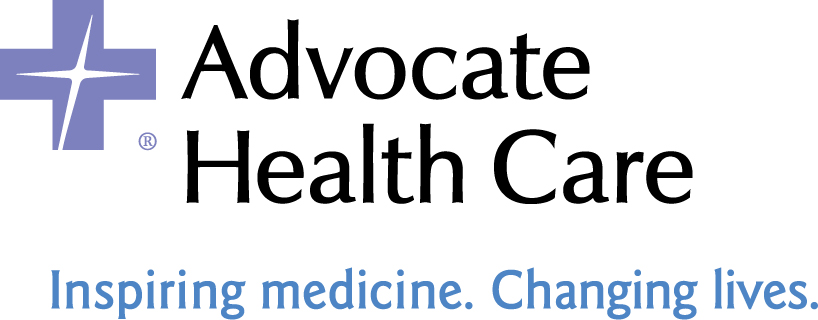
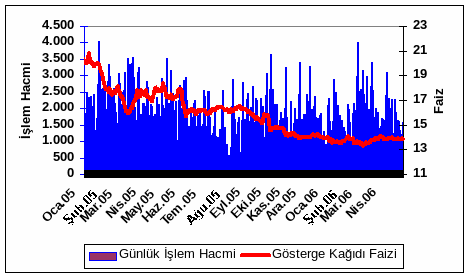
